Computer Vision Aided Blockage Prediction in Real-World Millimeter Wave Deployments
IEEE Global Communications Conference (GLOBECOM) Workshops 2022
Gouranga Charan and Ahmed Alkhateeb
Wireless Intelligence Lab, ASU
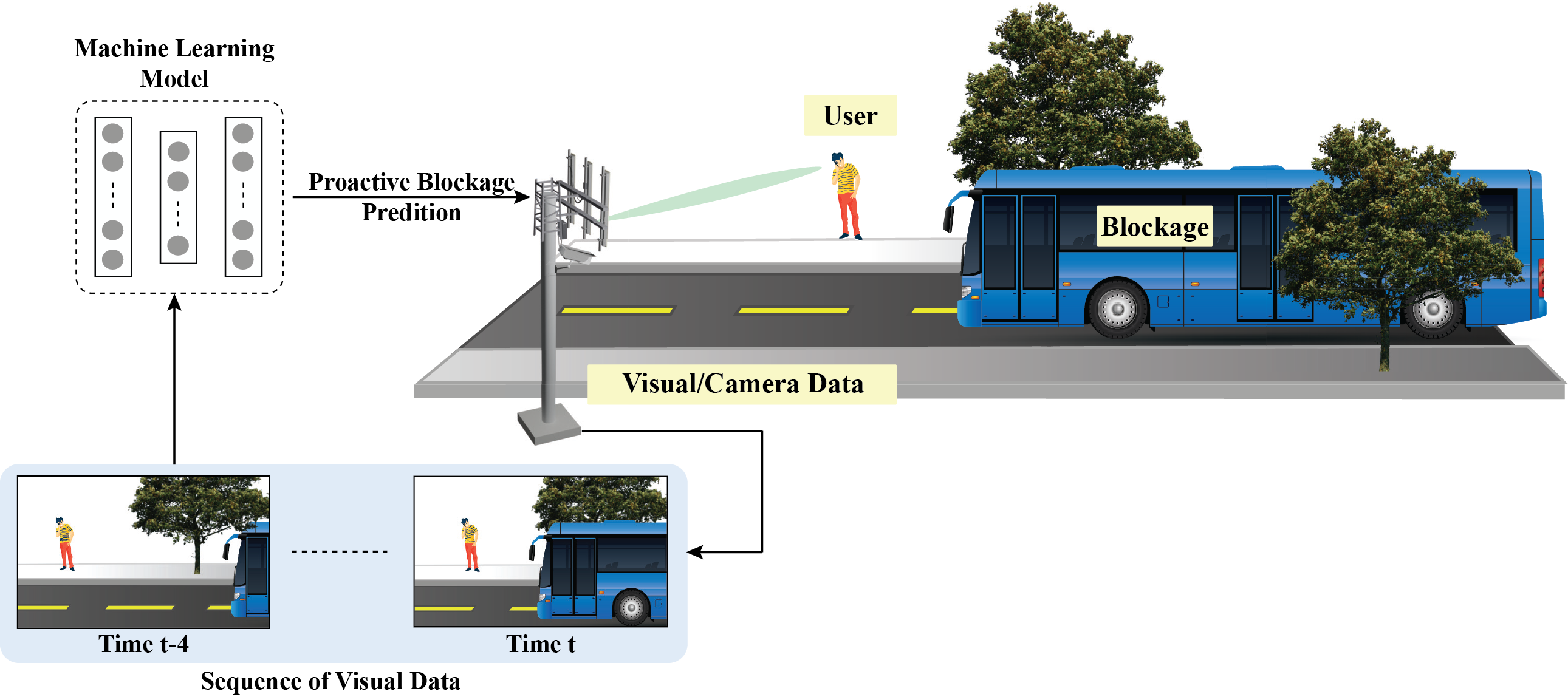
Given a sequence of images of the wireless environment, can the mmWave/sub-THz basestation proactively predict future line-of-sight (LOS) link blockages? Our machine learning-based solution can leverage the sequence of visual data to predict whether or not a future blockage will happen.
Abstract
This paper provides the first real-world evaluation of using visual (RGB camera) data and machine learning for proactively predicting millimeter wave (mmWave) dynamic link blockages before they happen. Proactively predicting line-of-sight (LOS) link blockages enables mmWave/sub-THz networks to make proactive network management decisions, such as proactive beam switching and hand-off) before a link failure happens. This can significantly enhance the network reliability and latency while efficiently utilizing the wireless resources. To evaluate this gain in reality, this paper (i) develops a computer vision-based solution that processes the visual data captured by a camera installed at the infrastructure node and (ii) studies the feasibility of the proposed solution based on the large-scale real-world dataset, DeepSense 6G, that comprises multi-modal sensing and communication data. Based on the adopted real-world dataset, the developed solution achieves ~90% accuracy in predicting blockages happening within the future 0.1s and ~80% for blockages happening within 1s, which highlights a promising solution for mmWave/sub-THz communication networks.
Proposed Solution
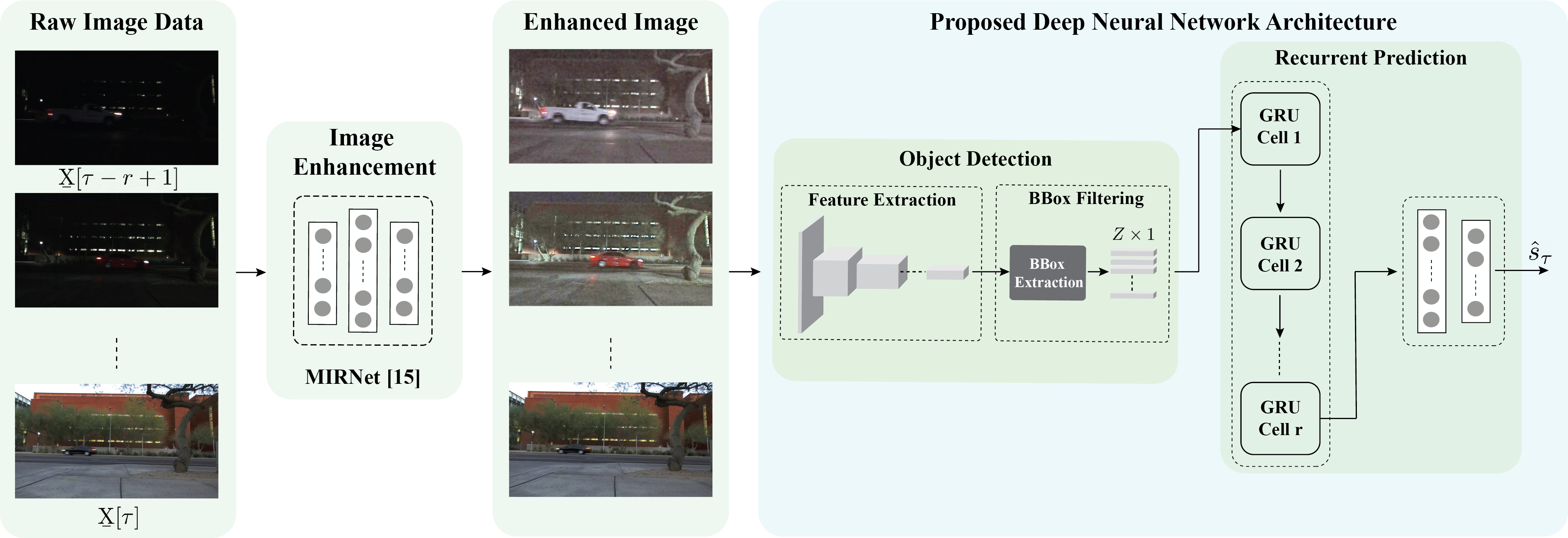
This figure presents the proposed architecture for vision-aided future blockage prediction. The raw visual data is first processed to enhance the images captured during night or low-light scenario. The processed sequence of images are then passed through the proposed deep neural network architecture consisting of a object detection model and recurrent neural network to predict the future blockage.
Video Presentation
DeepSense 6G Dataset
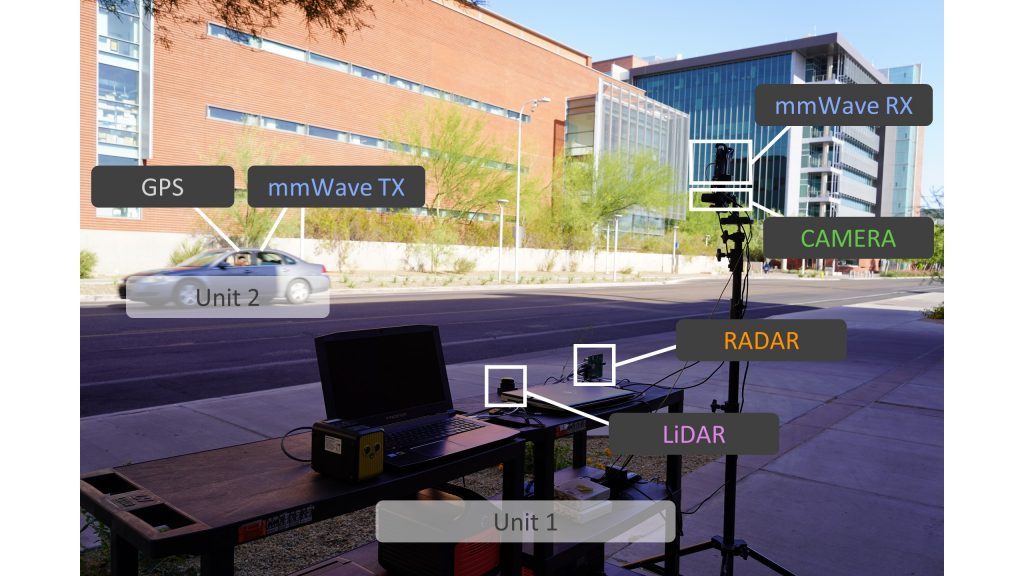
DeepSense 6G is a real-world multi-modal dataset that comprises coexisting multi-modal sensing and communication data, such as mmWave wireless communication, Camera, GPS data, LiDAR, and Radar, collected in realistic wireless environments. Link to the DeepSense 6G website is provided below.
Scenarios [17 -22]
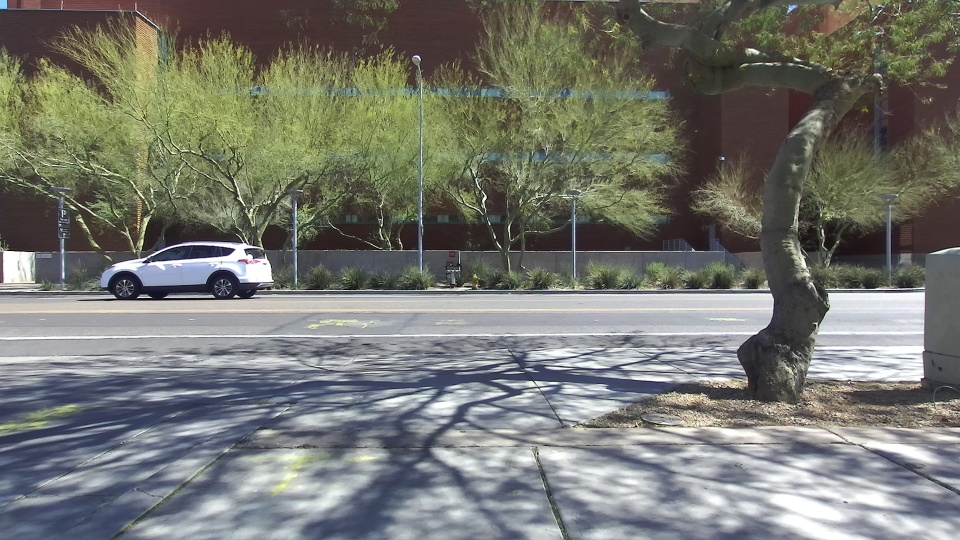
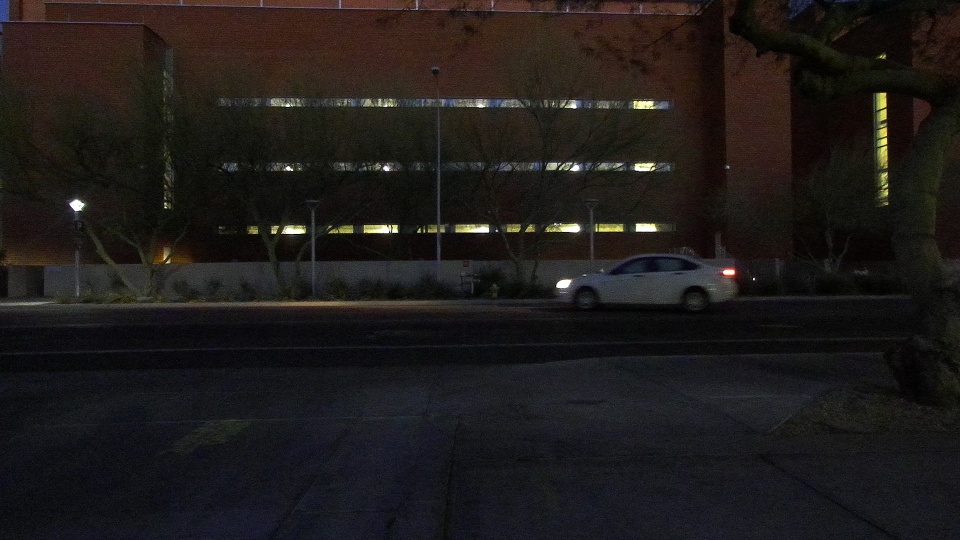
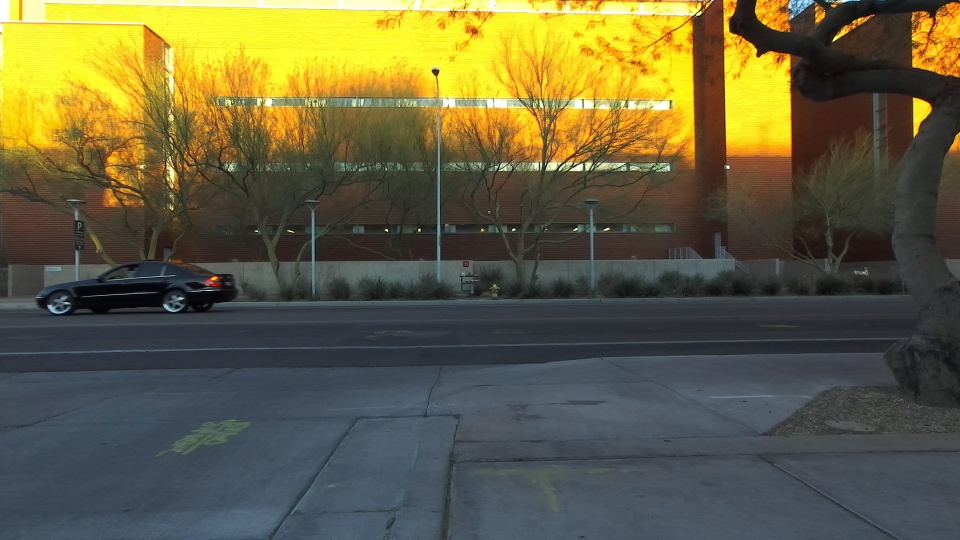
In this blockage prediction task, we build development/challenge datasets based on the DeepSense data from scenarios 17 – 22. For further details regarding the scenarios, follow the links provided below.
Citation
A. Alkhateeb, G. Charan, T. Osman, A. Hredzak, J. Morais, U. Demirhan, and N. Srinivas, “DeepSense 6G: A Large-Scale Real-World Multi-Modal Sensing and Communication Datasets,” IEEE Communications Magazine, 2023.
@Article{DeepSense,
author={Alkhateeb, Ahmed and Charan, Gouranga and Osman, Tawfik and Hredzak, Andrew and Morais, Joao and Demirhan, Umut and Srinivas, Nikhil},
title={{DeepSense 6G: A Large-Scale Real-World Multi-Modal Sensing and Communication Dataset}},
journal={IEEE Communications Magazine},
year={2023},
publisher={IEEE}}
G. Charan and A. Alkhateeb, “Computer Vision Aided Blockage Prediction in Real-World Millimeter Wave Deployments,” in submission to IEEE GLOBECOM, 2022
@INPROCEEDINGS{Charan2022,
author={Charan, Gouranga and Alkhateeb, Ahmed},
booktitle={2022 IEEE Globecom Workshops (GC Wkshps)},
title={Computer Vision Aided Blockage Prediction in Real-World Millimeter Wave Deployments},
year={2022},
pages={1711-1716},
doi={10.1109/GCWkshps56602.2022.10008524}}