Millimeter Wave V2V Beam Tracking using Radar:
Algorithms and Real-World Demonstration
Accepted to the European Signal Processing Conference (EUSIPCO), 2023
Hao Luo, Umut Demirhan, Ahmed Alkhateeb
Wireless Intelligence Lab, ASU
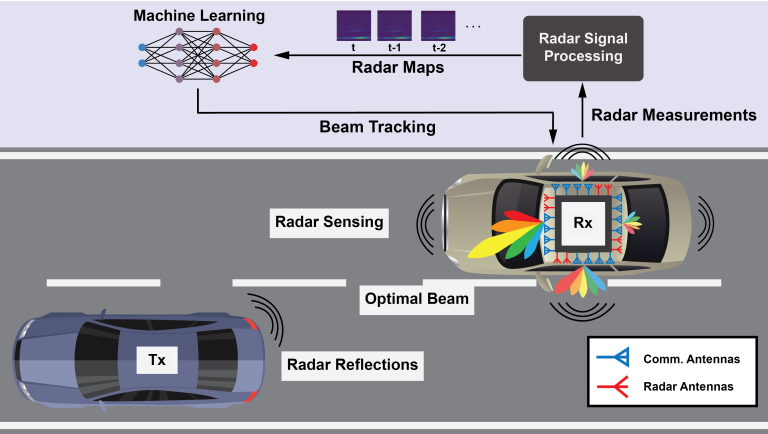
This figure presents the adopted V2V communication scenario, where the system model consists of a transmitter vehicle and a receiver vehicle. The transmitter employs a single omnidirectional antenna, while the receiver is equipped with four pairs of mmWave antenna arrays and FMCW radars in four separate directions. The receiver vehicle leverages the radar measurements to predict the optimal beam that communicates with the transmitter vehicle.
Abstract
Proposed Solution
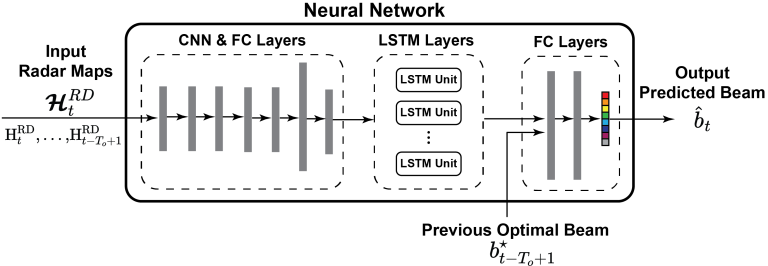
This figure presents the proposed end-to-end approach for radar-aided beam tracking. The range-Doppler maps, which are extracted from the radar measurements, are fed to the shown architecture. Before the beam prediction, the previous optimal beam index is combined with the output of the LSTM layers to provide the historical information of the transmitter.
Video Presentation
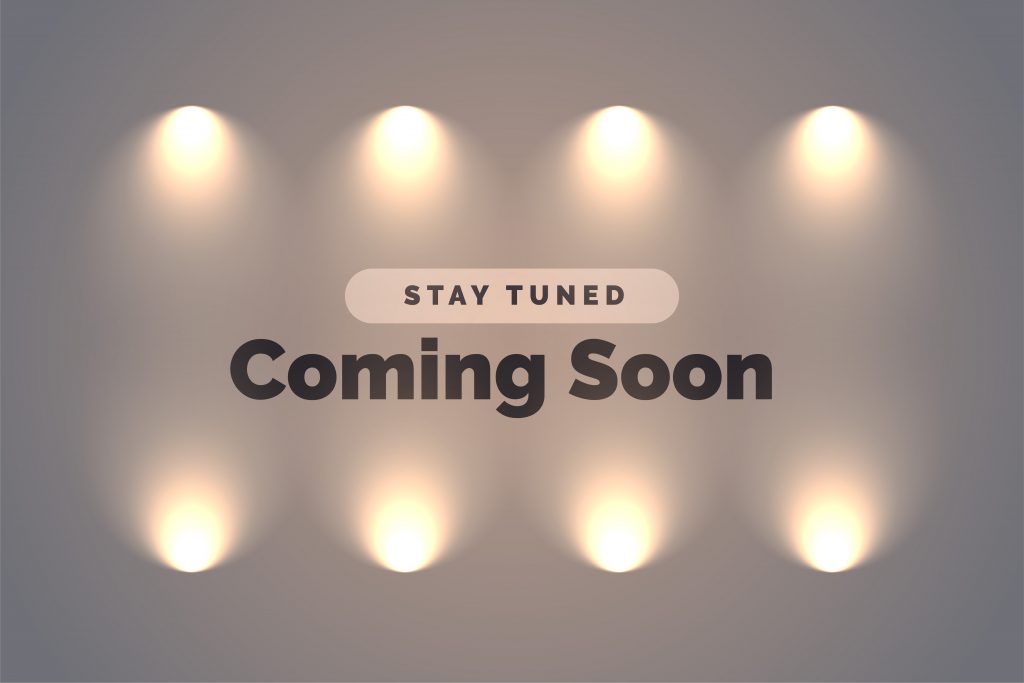
Reproducing the Results
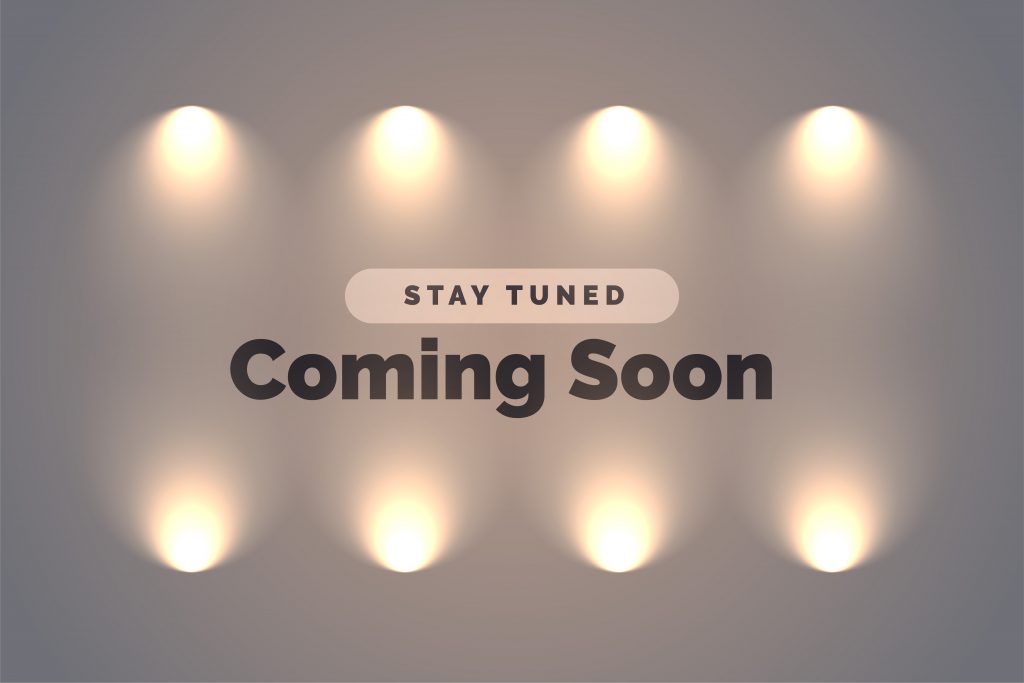
DeepSense 6G Dataset
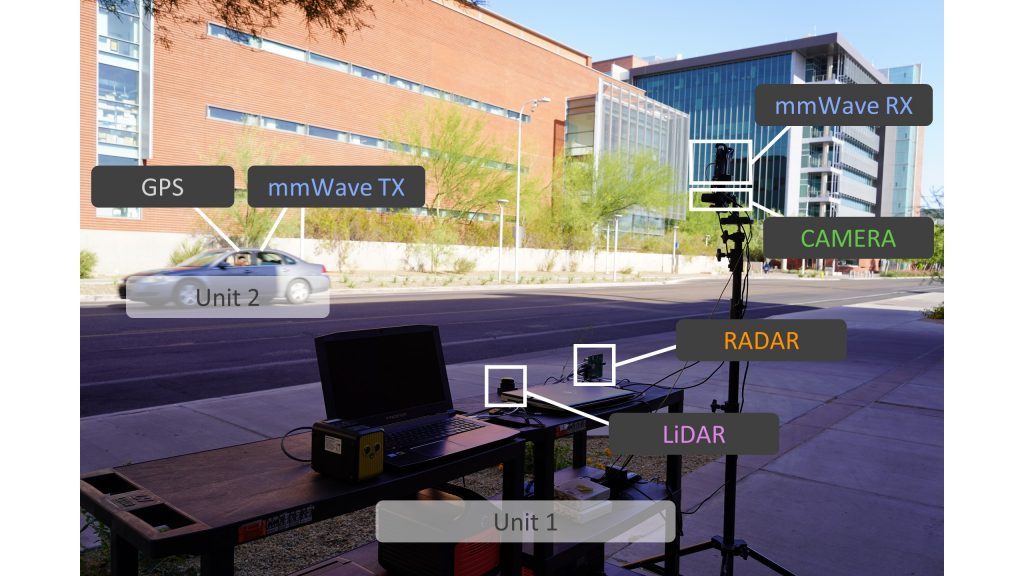
DeepSense 6G is a real-world multi-modal dataset that comprises coexisting multi-modal sensing and communication data, such as mmWave wireless communication, Camera, GPS data, LiDAR, and Radar, collected in realistic wireless environments. Link to the DeepSense 6G website is provided below.
Scenarios
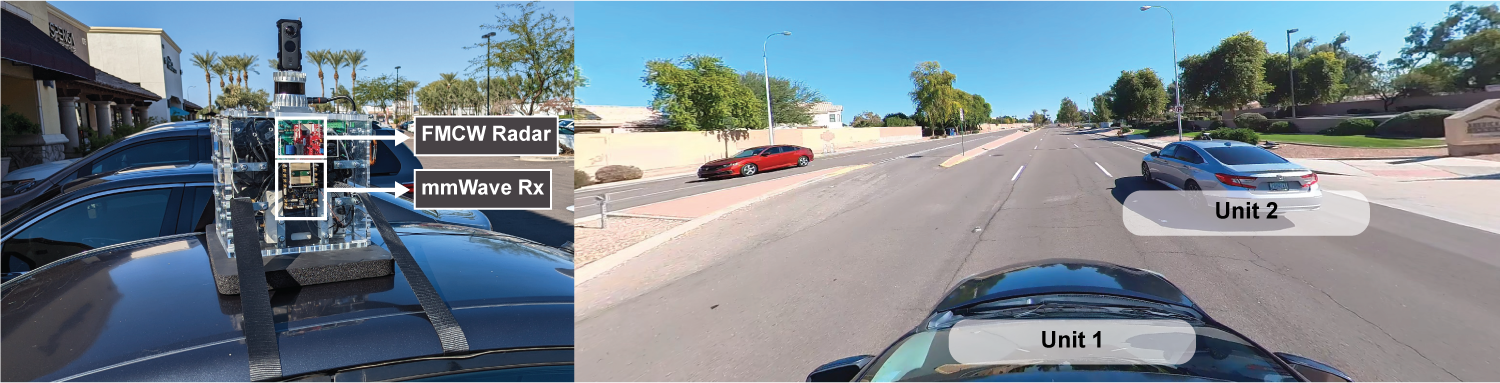
This figure presents the overview of the DeepSense 6G V2V testbed. The image on the left depicts the setup of the testbed, where mmWave antenna arrays and radars are placed on top of a vehicle (Unit 1). Four sets of mmWave receivers and radars are directed toward four sides of the box. The image on the right shows a sample from the data collection, where the transmitter vehicle (Unit 2) is equipped with an omnidirectional antenna.
Citation
@INPROCEEDINGS{Luo2023,
author={Luo, Hao and Demirhan, Umut and Alkhateeb, Ahmed},
booktitle={2023 31st European Signal Processing Conference (EUSIPCO)},
title={Millimeter Wave V2V Beam Tracking using Radar: Algorithms and Real-World Demonstration},
year={2023},
volume={},
number={},
pages={740-744},
doi={10.23919/EUSIPCO58844.2023.10289752}}
@Article{DeepSense,
author = {Alkhateeb, A. and Charan, G. and Osman, T. and Hredzak, A. and Morais, J. and Demirhan, U. and Srinivas, N.},
title = {{DeepSense 6G}: A Large-Scale Real-World Multi-Modal Sensing and Communication Dataset},
journal={IEEE Communications Magazine},
year = {2023},
pages={1-7},
doi={10.1109/MCOM.006.2200730}}