Vehicle Camera Guide mmWave Beams:
Approach and Real-world V2V Demonstration
Accepted to Asilomar Conference on Signals, Systems, and Computers(ACSSC), 2023
Tawfik Osman, Goranga Charan, Ahmed Alkhateeb
Wireless Intelligence Lab, ASU
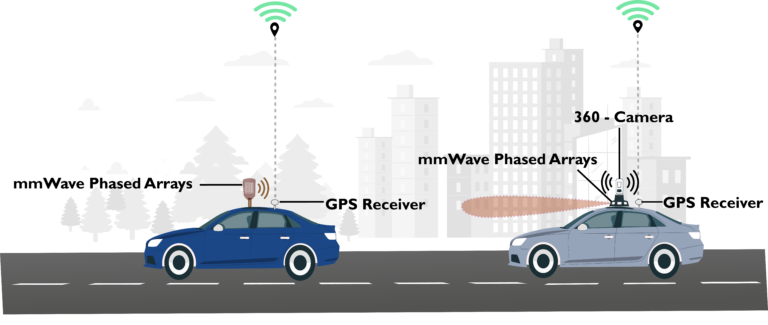
This figure presents the adopted V2V communication scenario, where the system model consists of a transmitter vehicle and a receiver vehicle. The transmitter employs a single omnidirectional antenna, while the receiver is equipped with four pairs of mmWave antenna arrays and off-the-shelf 360 camera device. The receiver vehicle leverages the camera measurements to predict the optimal beam that communicates with the transmitter vehicle.
Abstract
Accurately aligning millimeter-wave (mmWave) and terahertz (THz) narrow beams is essential to satisfy reliability and high data rates of 5G and beyond wireless communication systems. However, achieving this objective is difficult, especially in vehicle-to-vehicle (V2V) communication scenarios, where both transmitter and receiver are constantly mobile. Recently, additional sensing modalities, such as visual sensors, have attracted significant interest due to their capability to provide accurate information about the wireless environment. To that end, in this paper, we develop a deep learning solution for V2V scenarios to predict future beams using images from a 360 camera attached to the vehicle. The developed solution is evaluated on a real-world multi-modal mmWave V2V communication dataset comprising co-existing 360 camera and mmWave beam training data. The proposed vision-aided solution achieves ≈85% top-5 beam prediction accuracy while significantly reducing the beam training overhead. This highlights the potential of utilizing vision for enabling highly-mobile V2V communications.
Proposed Solution
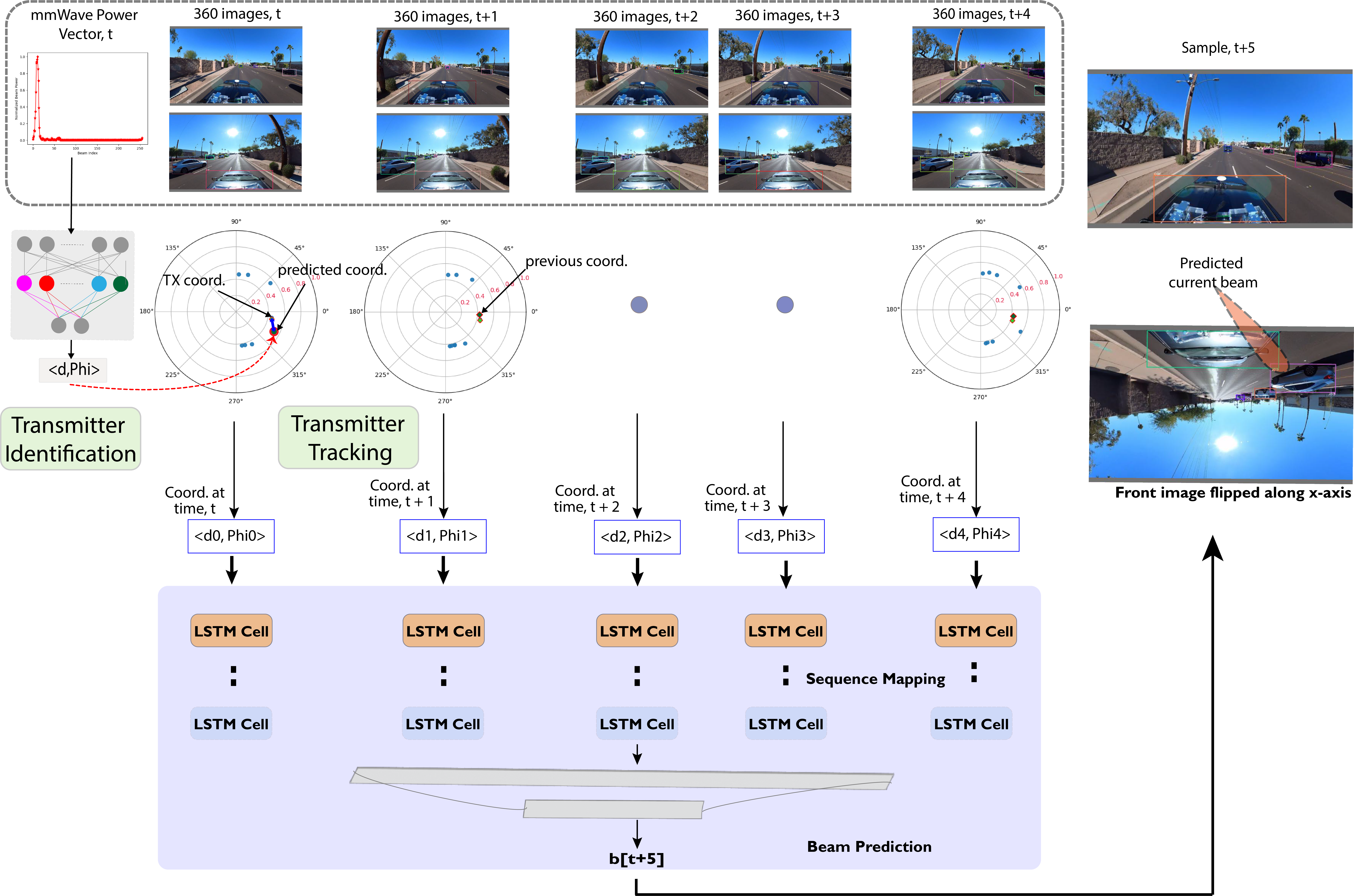
This figure presents an overview of the proposed deep learning and distance-based tracking solution. The bounding box coordinates for the front and back images are first transformed into a 2D polar plane. The mmWave beam power is fed into the transmitter identification model, to predict the current transmitter coordinates. The nearest distance-based algorithm is employed to track the transmitter coordinates in the 2D polar planes over the next four image samples. The tracked transmitter coordinates are fed to the LSTM and beam prediction sub-networks
Video Presentation
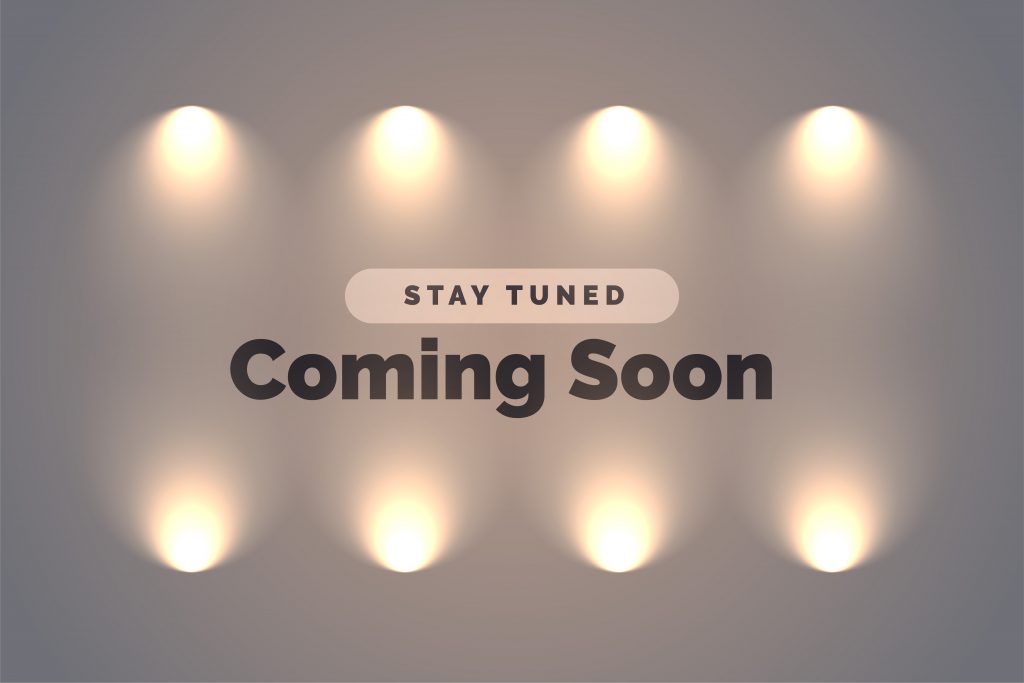
Reproducing the Results
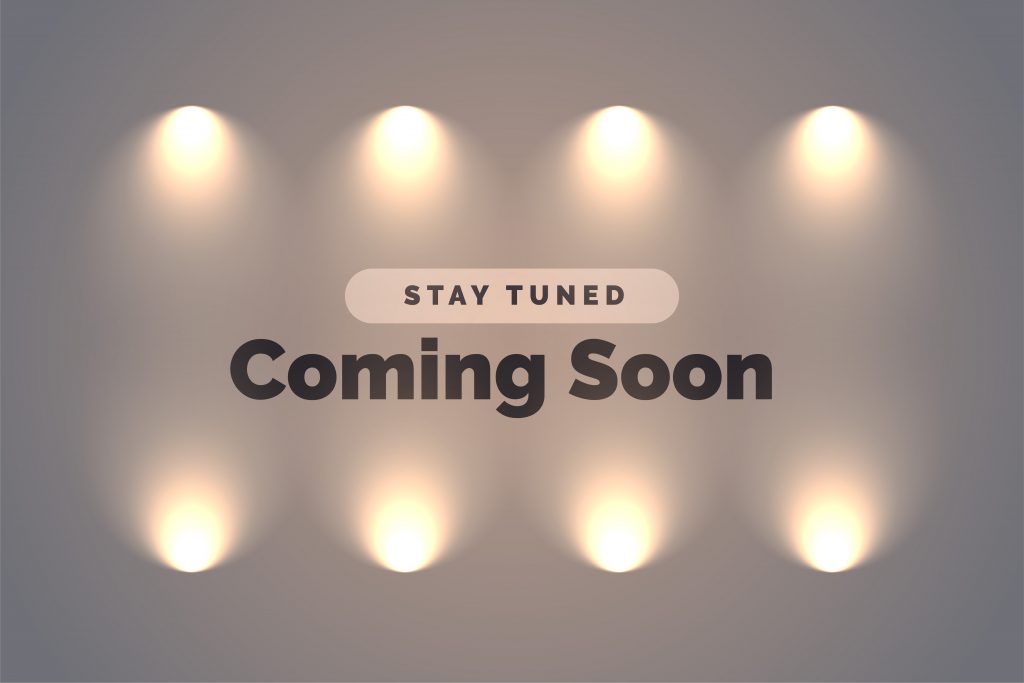
DeepSense 6G Dataset
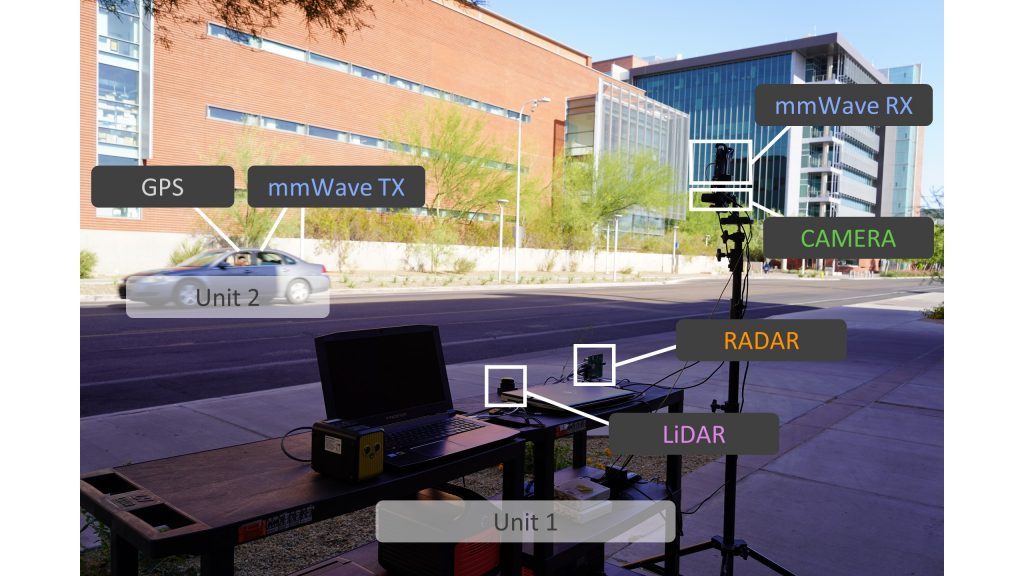
DeepSense 6G is a real-world multi-modal dataset that comprises coexisting multi-modal sensing and communication data, such as mmWave wireless communication, Camera, GPS data, LiDAR, and Radar, collected in realistic wireless environments. Link to the DeepSense 6G website is provided below.
Scenarios
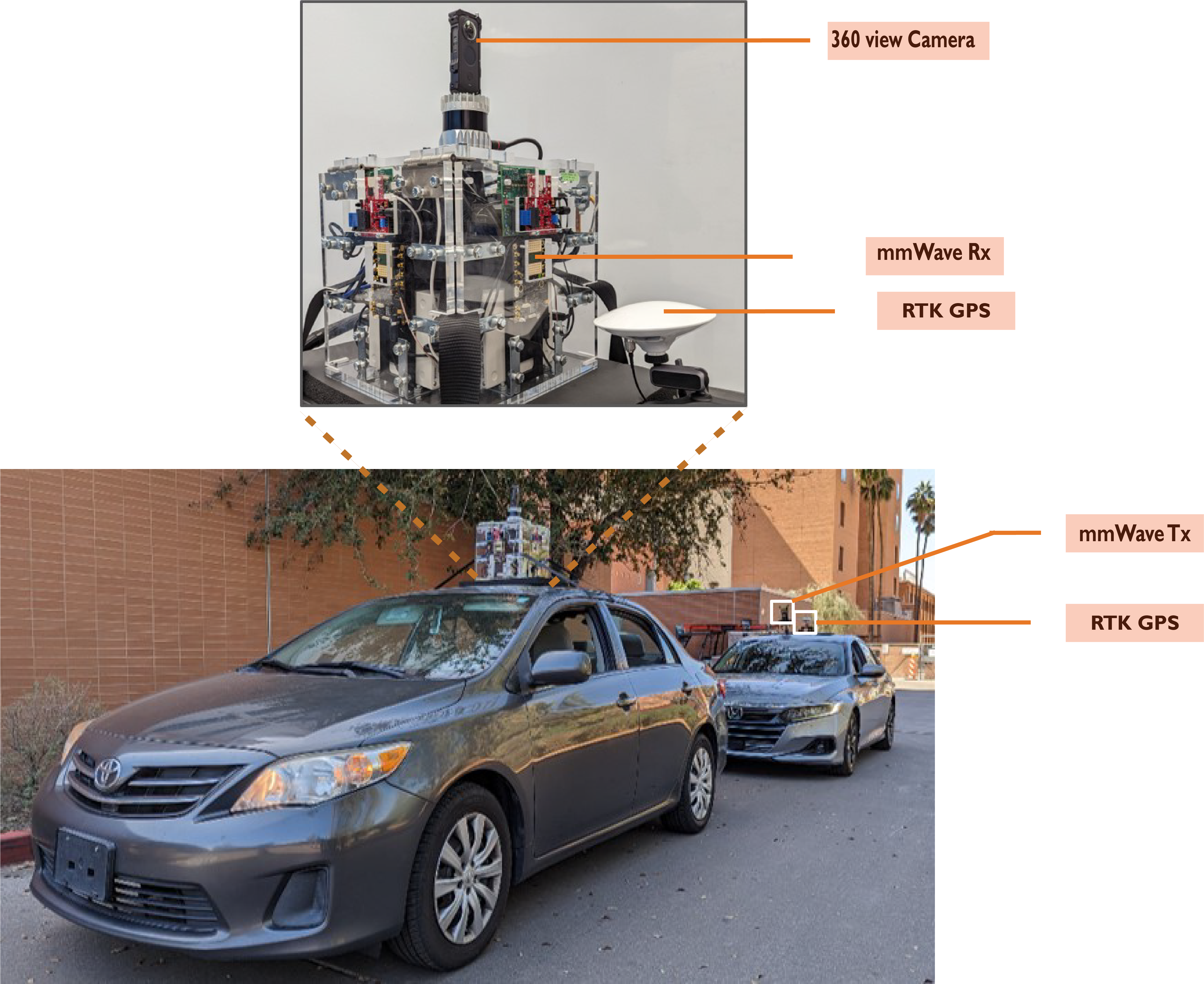
This figure shows a detailed description of the DeepSense 6G Testbed adopted to collect the real-world multi-modal V2V data samples. The front car (receiver unit) is equipped with four 60GHz mmWave Phased arrays, 360 degrees RGB camera, four mmWave FMCW radars, one 3D LiDAR, and a GPS receiver. The back car (transmitter unit) is equipped with a 60 GHz quasi-omni antennas and a GPS receiver.
Citation
T.Osman, G. Charan and A. Alkhateeb, “Vehicle Cameras Guide mmWave Beams: Approach and Real-World V2V Demonstration”, accepted to Asilomar Conference on Signals, Systems, and Computers (ACSSC 2023).
@misc{osman2023vehicle,
title={Vehicle Cameras Guide mmWave Beams: Approach and Real-World V2V Demonstration},
author={Tawfik Osman and Gouranga Charan and Ahmed Alkhateeb},
year={2023},
eprint={2308.10362},
archivePrefix={arXiv},
primaryClass={cs.IT}
}
@Article{DeepSense,
author = {Alkhateeb, A. and Charan, G. and Osman, T. and Hredzak, A. and Morais, J. and Demirhan, U. and Srinivas, N.},
title = {{DeepSense 6G}: A Large-Scale Real-World Multi-Modal Sensing and Communication Dataset},
journal={IEEE Communications Magazine},
year = {2023},
pages={1-7},
doi={10.1109/MCOM.006.2200730}}