Sensing Aided Reconfigurable Intelligent Surfaces for 3GPP 5G Transparent Operation
Shuaifeng Jiang1, Ahmed Hindy2, and Ahmed Alkhateeb1
1Wireless Intelligence Lab, ASU
2Motorola Mobility LLC (a Lenovo company)
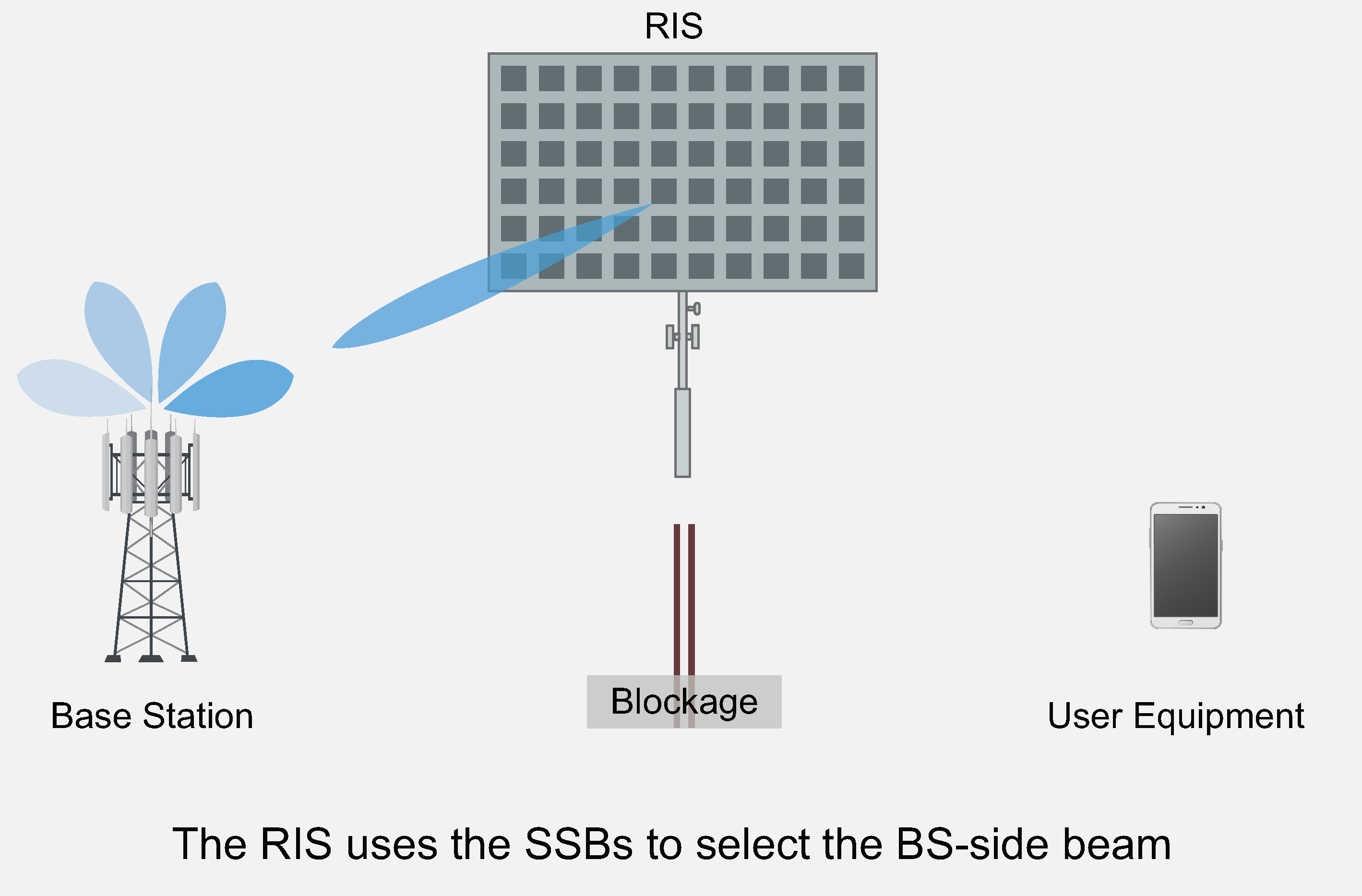
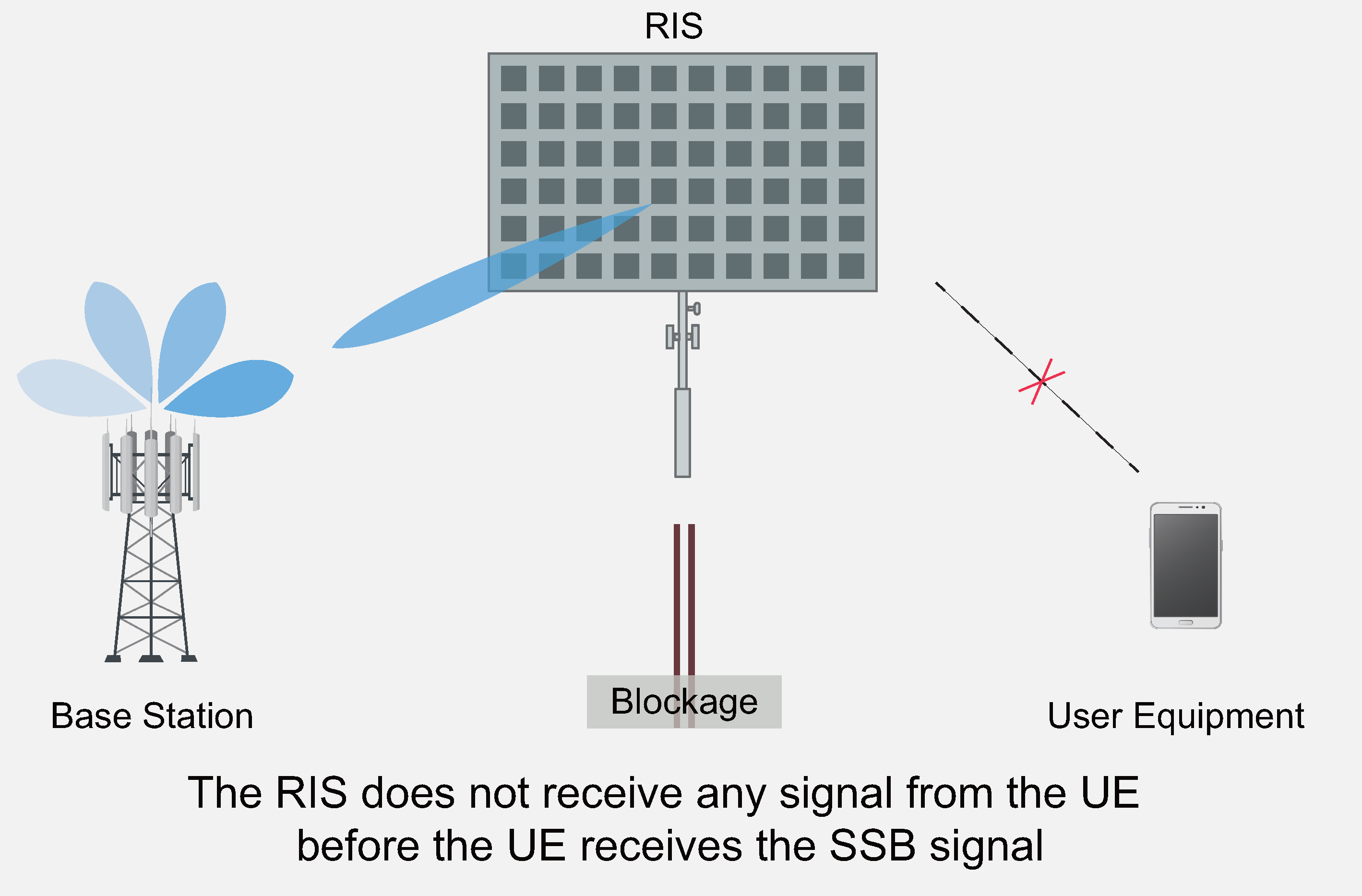
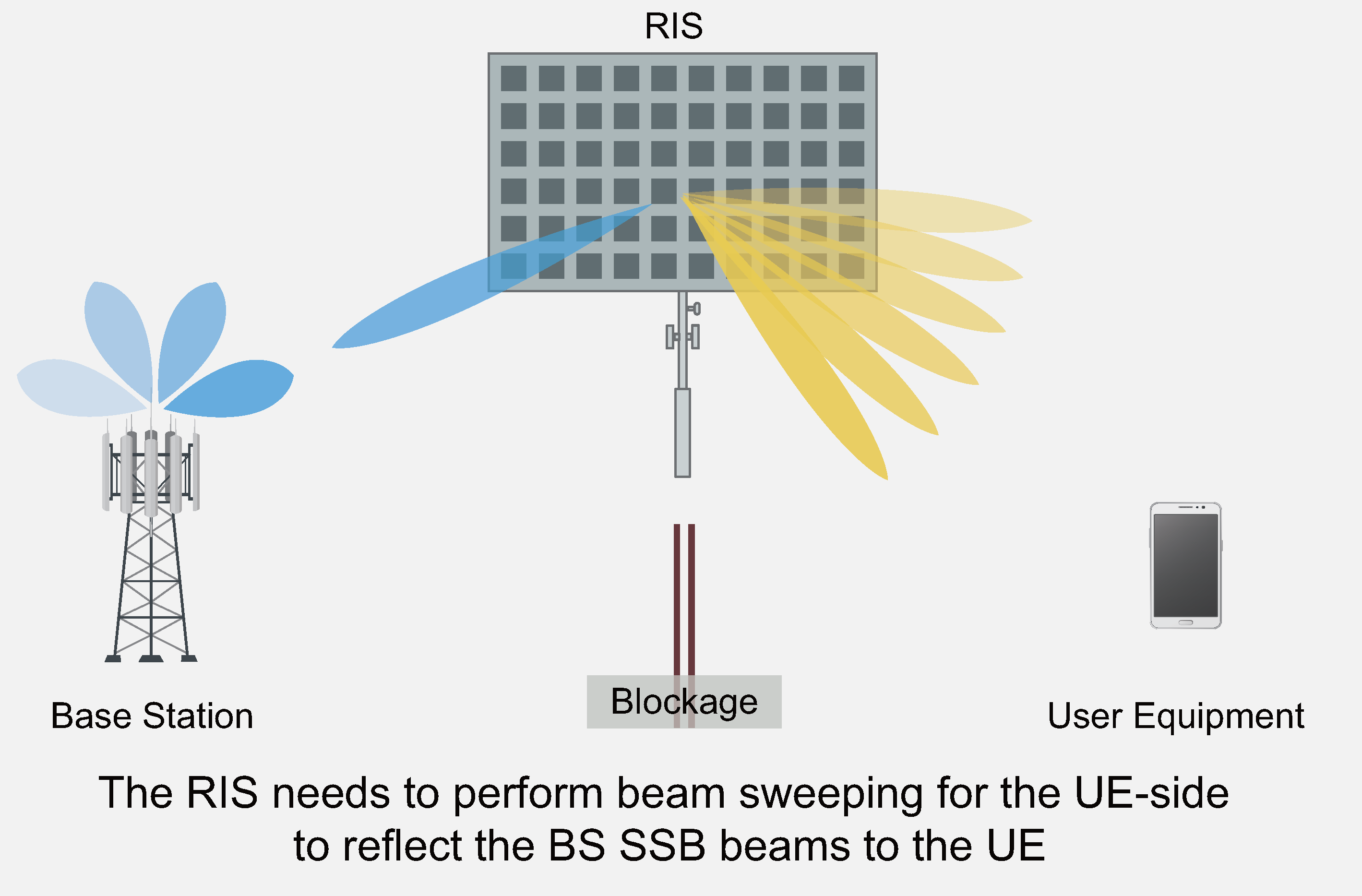
This figure presents the key challenge in applying the 3GPP 5G initial access process for an RIS-aided communication system; the UE only sends a preamble sequence after it receives and decodes the SSBs. Hence, the RIS cannot have any information about the UE channel and it needs to perform beam sweeping over a very large codebook. In this work, we utilize sensing to enable transparent RIS beam selection with reduced beam training overhead.
Abstract
Can reconfigurable intelligent surfaces (RISs) operate in a standalone mode that is completely transparent to the 3GPP 5G initial access process? Realizing that may greatly simplify the deployment and operation of these surfaces and reduce the infrastructure control overhead. This paper investigates the feasibility of building standalone/transparent RIS systems and shows that one key challenge lies in determining the user equipment (UE)-side RIS beam reflection direction. To address this challenge, we propose to equip the RISs with multi-modal sensing capabilities (e.g., using wireless and visual sensors) that enable them to develop some perception of the surrounding environment and the mobile users. Based on that, we develop a machine learning framework that leverages the wireless and visual sensors at the RIS to select the high-performance beams between the base station (BS) and UEs and enable standalone/transparent RIS operation for 5G high-frequency systems. Using a high-fidelity synthetic dataset with co-existing wireless and visual data, we extensively evaluate the performance of the proposed framework. Experimental results demonstrate that the proposed approach can accurately predict the BS and UE-side candidate beams, and that the standalone RIS beam selection solution is capable of realizing near-optimal achievable rates with significantly reduced beam training overhead.
Proposed Solution
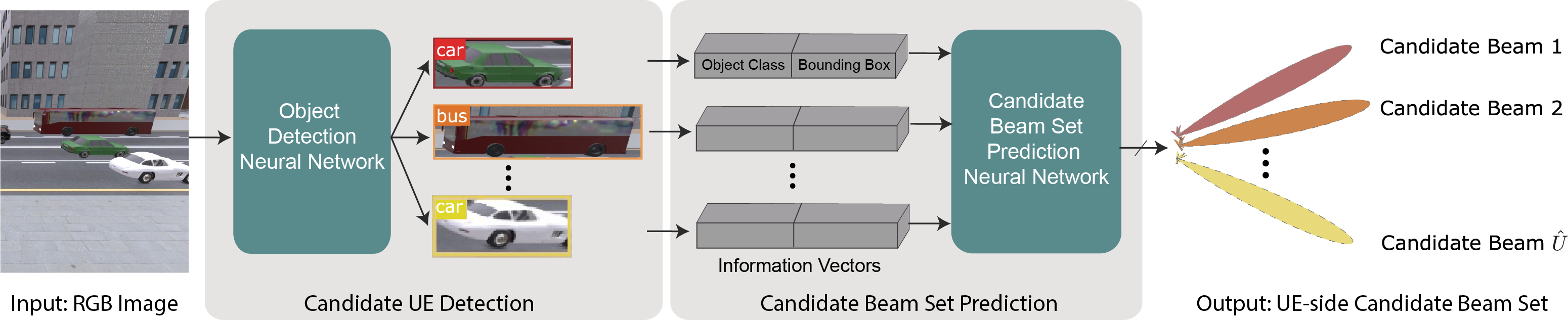
The figure illustrates the proposed ML framework for the RIS UE-side candidate beam set prediction. Given the visual information of the surrounding environment of the RIS, the framework first detects the candidate UEs with an object detector. Then the information about the candidate UEs (bounding boxes and object classes) are used to predict the candidate beams.
DeepSense 6G Dataset
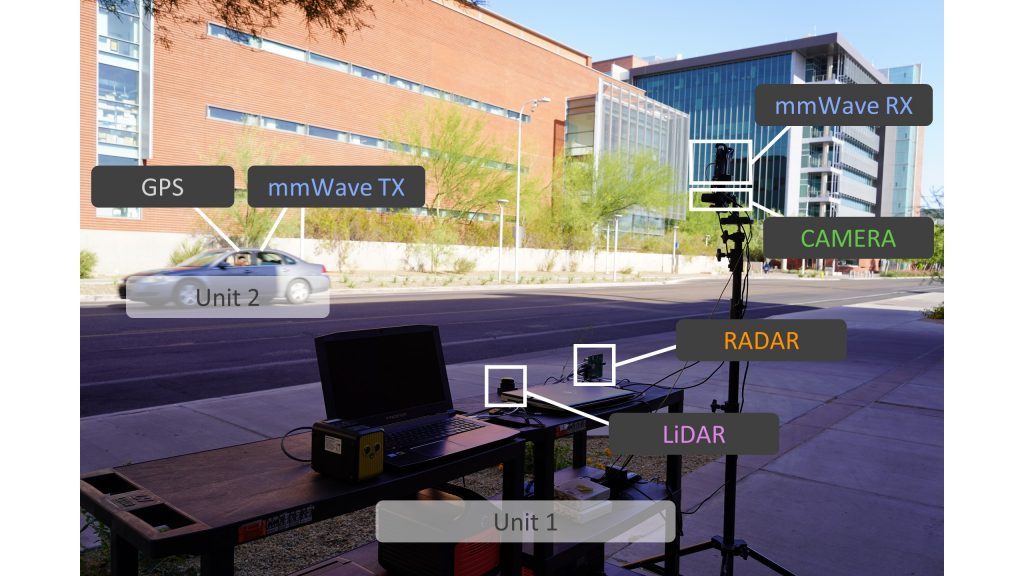
DeepSense 6G is a real-world multi-modal dataset that comprises coexisting multi-modal sensing and communication data, such as mmWave wireless communication, Camera, GPS data, LiDAR, and Radar, collected in realistic wireless environments. Link to the DeepSense 6G website is provided below.
Scenario
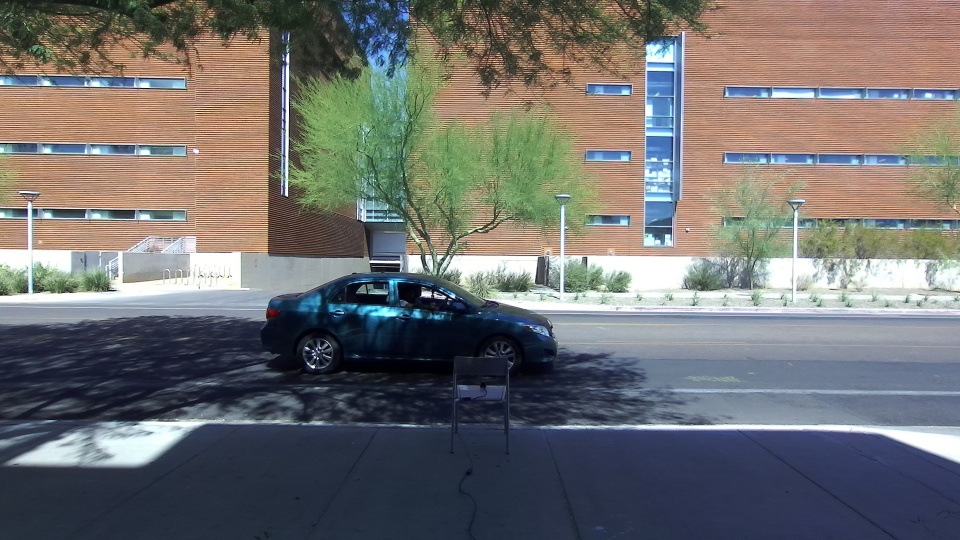
In this beam tracking task, we build development/challenge datasets based on the DeepSense data from scenario 8. For further details regarding the scenarios, follow the links provided below.
Reproducing the Results
Citation
A. Alkhateeb, G. Charan, T. Osman, A. Hredzak, J. Morais, U. Demirhan, and N. Srinivas, “DeepSense 6G: A Large-Scale Real-World Multi-Modal Sensing and Communication Datasets,” to be available on arXiv, 2022. [Online]. Available: https://www.DeepSense6G.net
@Article{DeepSense,
author = {Alkhateeb, A. and Charan, G. and Osman, T. and Hredzak, A. and Morais, J. and Demirhan, U. and Srinivas, N.},
title = {{DeepSense 6G}: A Large-Scale Real-World Multi-Modal Sensing and Communication Dataset},
journal={to be available on arXiv},
year = {2022},
url = {https://www.DeepSense6G.net},}
@Article{jiang2023sensing,
title={Sensing Aided Reconfigurable Intelligent Surfaces for 3GPP 5G Transparent Operation},
author={Jiang, Shuaifeng and Hindy, Ahmed and Alkhateeb, Ahmed},
journal={IEEE Transactions on Communications},
year={2023},
publisher={IEEE}}