LiDAR Aided Future Beam Prediction in Real-World Millimeter Wave V2I Communications
Shuaifeng Jiang1, Gouranga Charan1, and Ahmed Alkhateeb1
1Wireless Intelligence Lab, ASU
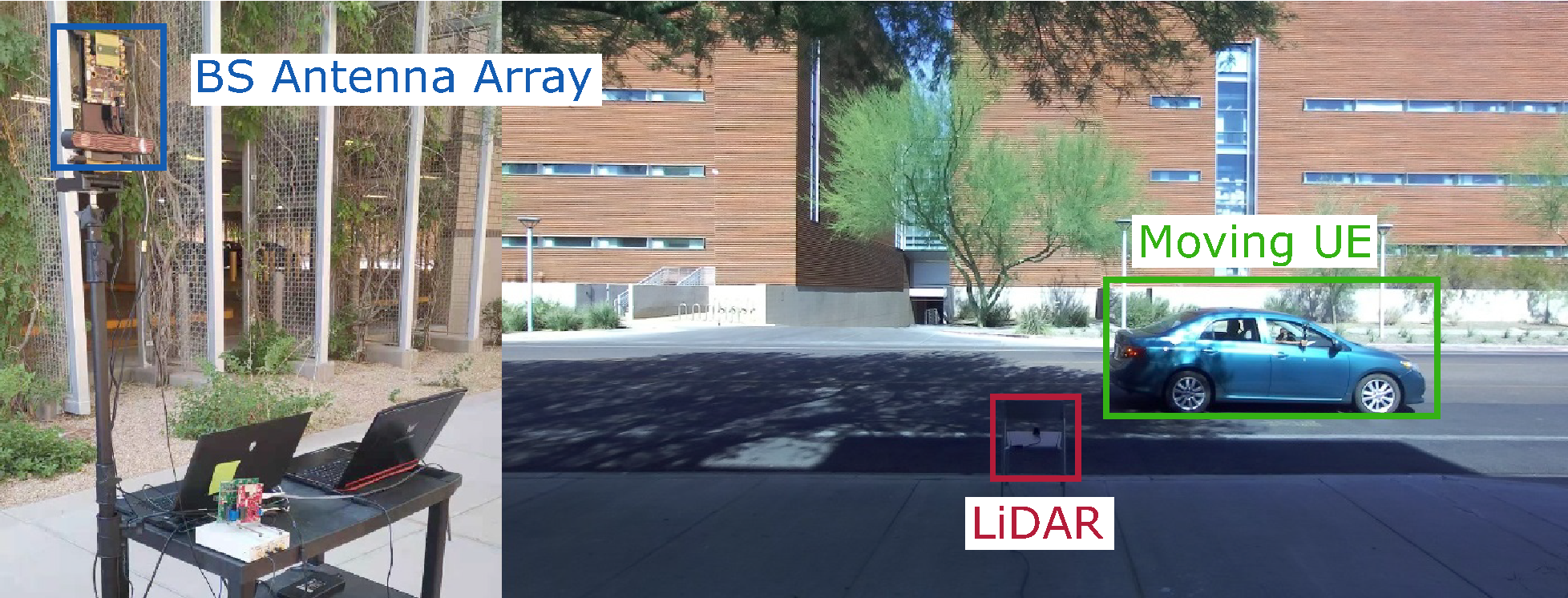
Using the LiDAR sensing information of the wireless environment, can the mmWave/sub-THz base station predict the optimal beam index?
Our machine learning-based solution can exploit such sensing capability to efficiently guide mmWave/THz beam prediction.
Abstract
This paper presents the first large-scale real-world evaluation for using LiDAR data to guide the mmWave beam prediction task. A machine learning (ML) model that leverages the LiDAR sensory data to predict the current and future beams was developed. Based on the large-scale real-world dataset, DeepSense 6G, this model was evaluated in a vehicle-to-infrastructure communication scenario with highly-mobile vehicles. The experimental results show that the developed LiDAR-aided beam prediction and tracking model can predict the optimal beam in 95% of the cases and with more than 90% reduction in the beam training overhead. The LiDAR-aided beam tracking achieves comparable accuracy performance to a baseline solution that has perfect knowledge of the previous optimal beams, without requiring any knowledge about the previous optimal beam information and without any need for beam calibration. This highlights a promising solution for the critical beam alignment challenges in mmWave and terahertz communication systems.
Proposed Solution

This figure presents the proposed framework for LiDAR-aided beam prediction. The BS senses the environment and the moving UE with a LiDAR. The sensing information is then processed by a deep learning model to predict optimal beams for the current and future time steps at the base station.
DeepSense 6G Dataset
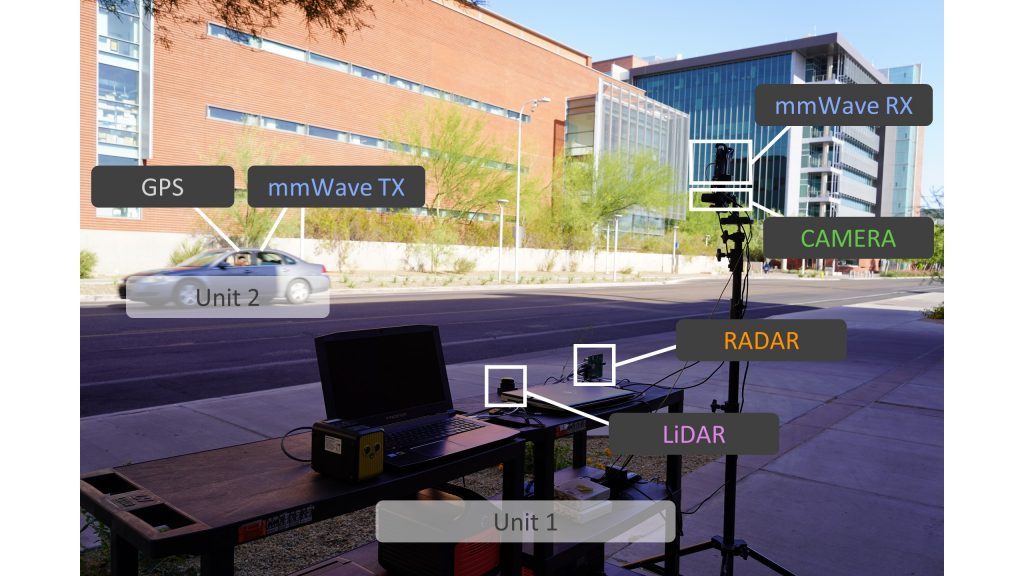
DeepSense 6G is a real-world multi-modal dataset that comprises coexisting multi-modal sensing and communication data, such as mmWave wireless communication, Camera, GPS data, LiDAR, and Radar, collected in realistic wireless environments. Link to the DeepSense 6G website is provided below.
Scenario
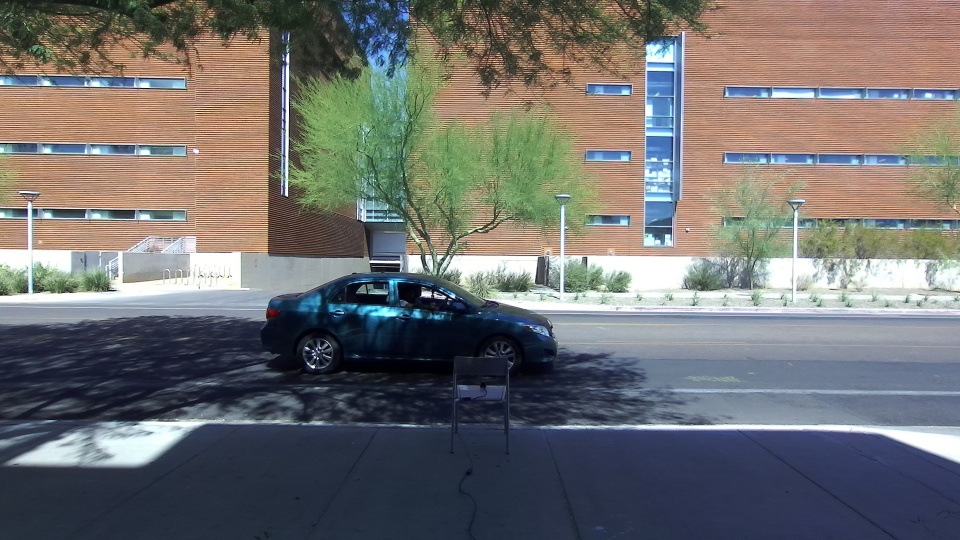
In this beam tracking task, we build development/challenge datasets based on the DeepSense data from scenario 8. For further details regarding the scenarios, follow the links provided below.
Reproducing the Results
Citation
A. Alkhateeb, G. Charan, T. Osman, A. Hredzak, J. Morais, U. Demirhan, and N. Srinivas, “DeepSense 6G: A Large-Scale Real-World Multi-Modal Sensing and Communication Datasets,” to be available on arXiv, 2022. [Online]. Available: https://www.DeepSense6G.net
@Article{DeepSense,
author = {Alkhateeb, A. and Charan, G. and Osman, T. and Hredzak, A. and Morais, J. and Demirhan, U. and Srinivas, N.},
title = {{DeepSense 6G}: A Large-Scale Real-World Multi-Modal Sensing and Communication Dataset},
journal={to be available on arXiv},
year = {2022},
url = {https://www.DeepSense6G.net},}
S. Jiang, G. Charan, and A. Alkhateeb, “LiDAR Aided Future Beam Prediction in Real-World Millimeter Wave V2I Communications.” in IEEE Wireless Communications Letters, 2022.
@Article{jiang2022lidar,
author = {Jiang, Shuaifeng and Charan, Gouranga and Alkhateeb, Ahmed},
title = {{LiDAR Aided Future Beam Prediction in Real-World Millimeter Wave V2I Communications}},
journal={IEEE Wireless Communications Letters},
year = {2022},}