LiDAR-Aided Mobile Blockage Prediction in Real-World mmWave Systems
Fig 1. a mmWave/sub-THz basestation leverages a LiDAR sensor to provide environment awareness and enable proactive link blockage prediction. The adopted neural network model is also shown, which consists of convolutional and prediction components.
Key ideas
- A typical LiDAR sensor sends pulsed light waves into the surrounding environment. These pulses are reflected by the objects and returned to the sensor; and the sensor uses the round-trip time to calculate the distance it traveled
- By sending and receiving laser beams, the LiDAR sensor collects a 2-D point cloud map of the surrounding environment
- We propose to leverage these LiDAR sensory data to detect if an object is going to block the mmWave communication link between the basestation and the mobile user.
Applications
- Proactively manage hand-off/beam-switching decisions
- Reduce initial access latency, increase reliability and resource utilization efficiency of future mmWave/THz networks
More information about this research direction
Paper: Shunyao Wu, Chaitali Chakrabarti, and Ahmed Alkhateeb, “LiDAR-Aided Mobile Blockage Prediction in Real-World Millimeter Wave Systems,” arXiv preprint arXiv:2111.09581 (2021).
Abstract: Line-of-sight link blockages represent a key challenge for the reliability and latency of millimeter wave (mmWave) and terahertz (THz) communication networks. This paper proposes to leverage LiDAR sensory data to provide awareness about the communication environment and proactively predict dynamic link blockages before they happen. This allows the network to make proactive decisions for hand-off/beam switching which enhances its reliability and latency. We formulate the LiDAR-aided blockage prediction problem and present the first real-world demonstration for LiDAR-aided blockage prediction in mmWave systems. In particular, we construct a large-scale real-world dataset, based on the DeepSense 6G structure, that comprises co-existing LiDAR and mmWave communication measurements in outdoor vehicular scenarios. Then, we develop an efficient LiDAR data denoising (static cluster removal) algorithm and a machine learning model that proactively predicts dynamic link blockages. Based on the real-world dataset, our LiDAR-aided approach is shown to achieve 95% accuracy in predicting blockages happening within 100ms and more than 80% prediction accuracy for blockages happening within one second. If used for proactive hand-off, the proposed solutions can potentially provide an order of magnitude saving in the network latency, which highlights a promising direction for addressing the blockage challenges in mmWave/sub-THz networks.
@misc{Wu2021,
title={LiDAR-Aided Mobile Blockage Prediction in Real-World Millimeter Wave Systems},
author={Wu, Shunyao and Chakrabarti, Chaitali and Alkhateeb, Ahmed},
year={2021},
eprint={2111.09581},
archivePrefix={arXiv},
primaryClass={eess.SP}}
To reproduce the results in this paper:
Simulation codes:
Coming soon
These simulations use the DeepSense scenarios:
Scenario "x"
Example: Steps to generate the results in this figure
- coming soon
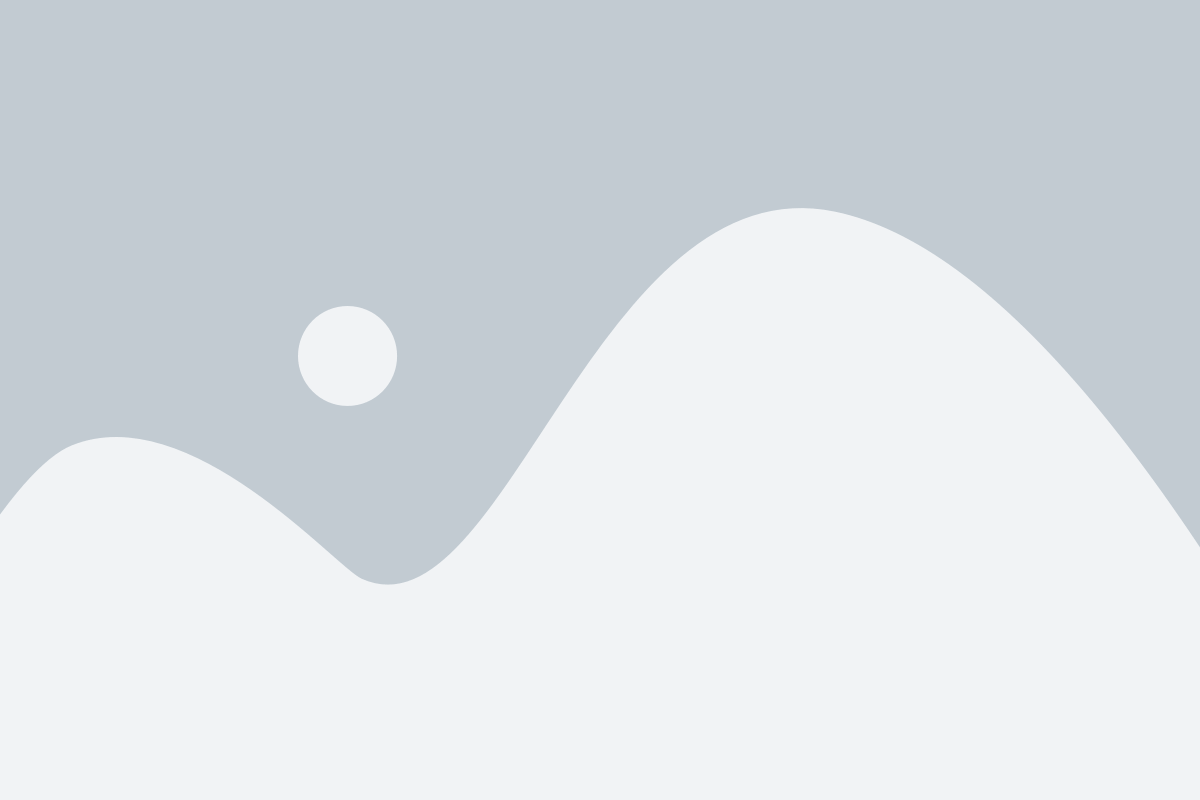