LiDAR-Aided Mobile Blockage Prediction in Real-World Millimeter Wave Systems
IEEE Open Journal of the Communications Society ( Volume: 3)
Shunyao Wu, Chaitali Chakrabarti, and Ahmed Alkhateeb
Wireless Intelligence Lab
Arizona State University
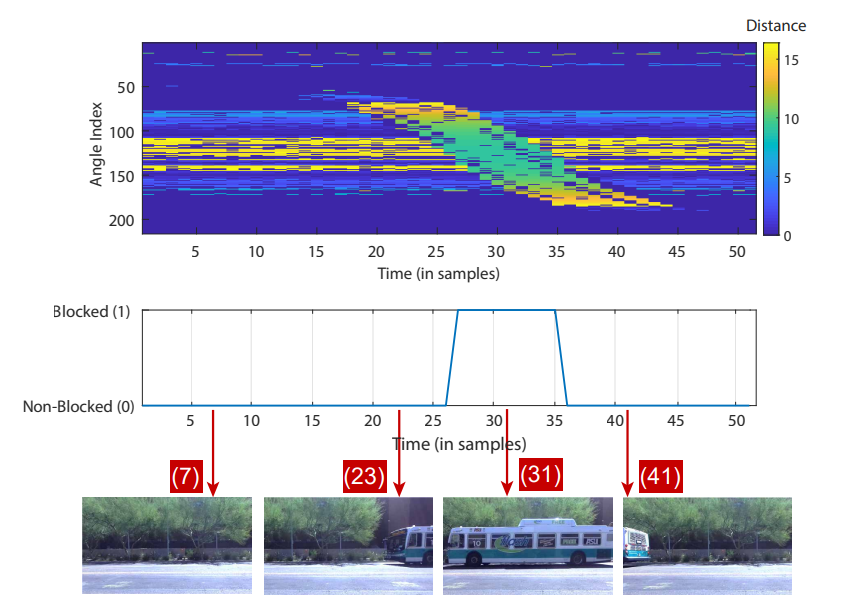
Given a sequence of LiDAR samples, can the basestation proactively predict future line-of-sight (LOS) link blockages? Our machine learning-based solution can leverage the sequence of processed LiDAR samples to predict whether or not a future blockage will happen.
Abstract
Line-of-sight link blockages represent a key challenge for the reliability and latency of millimeter wave (mmWave) and terahertz (THz) communication networks. This paper proposes to leverage LiDAR sensory data to provide awareness about the communication environment and proactively predict dynamic link blockages before they happen. This allows the network to make proactive decisions for handoff/beam switching which enhances its reliability and latency. We formulate the LiDAR-aided blockage prediction problem and present the first real-world demonstration for LiDARaided blockage prediction in mmWave systems. In particular, we construct a large-scale real-world dataset, based on the DeepSense 6G structure, that comprises co-existing LiDAR and mmWave communication measurements in outdoor vehicular scenarios. Then, we develop an efficient LiDAR data denoising (static cluster removal) algorithm and a machine learning model that proactively predicts dynamic link blockages. Based on the real-world dataset, our LiDAR-aided approach is shown to achieve 95% accuracy in predicting blockages happening within 100ms and more than 80% prediction accuracy for blockages happening within one second. If used for proactive hand-off, the proposed solutions can potentially provide an order of magnitude saving in the network latency, which highlights a promising direction for addressing the blockage challenges in mmWave/sub-THz networks.
Proposed Solution
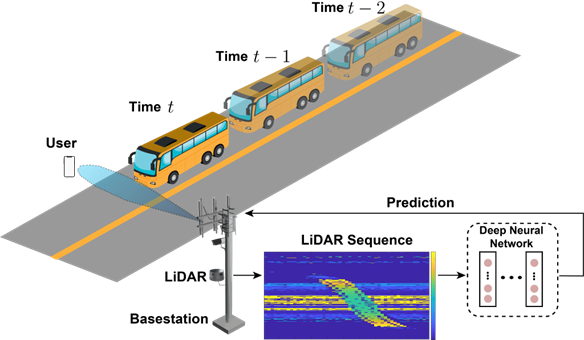
This figure illustrates the overall system model where a mmWave/sub-THz basestation leverages a LiDAR sensor to provide environmental awareness and enable the proposed proactive link blockage prediction approach.
Video Presentation
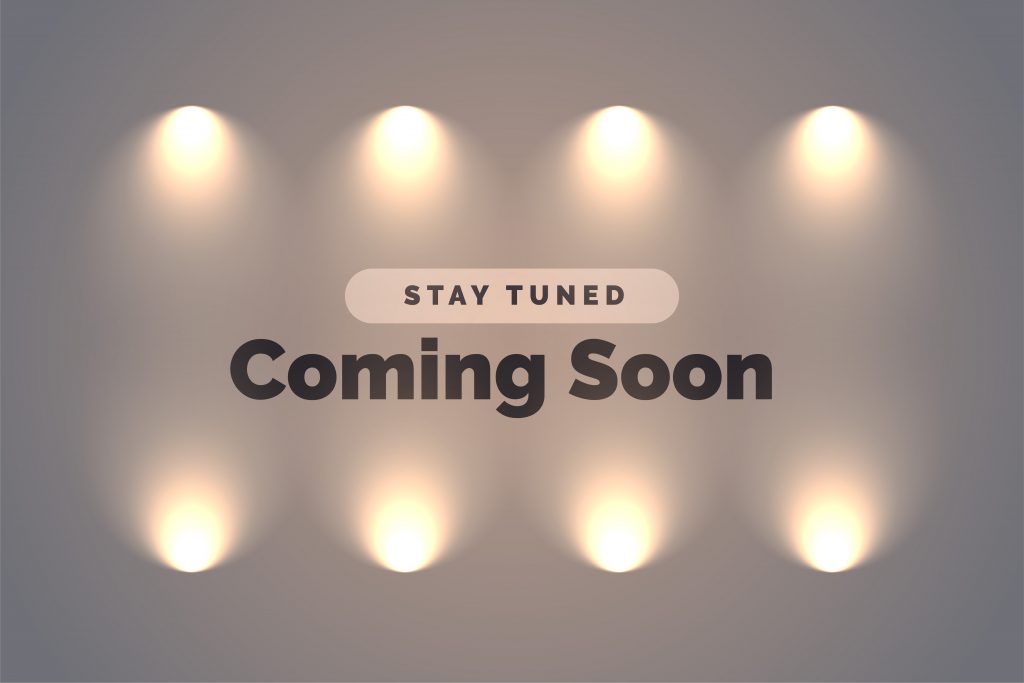
DeepSense 6G Dataset
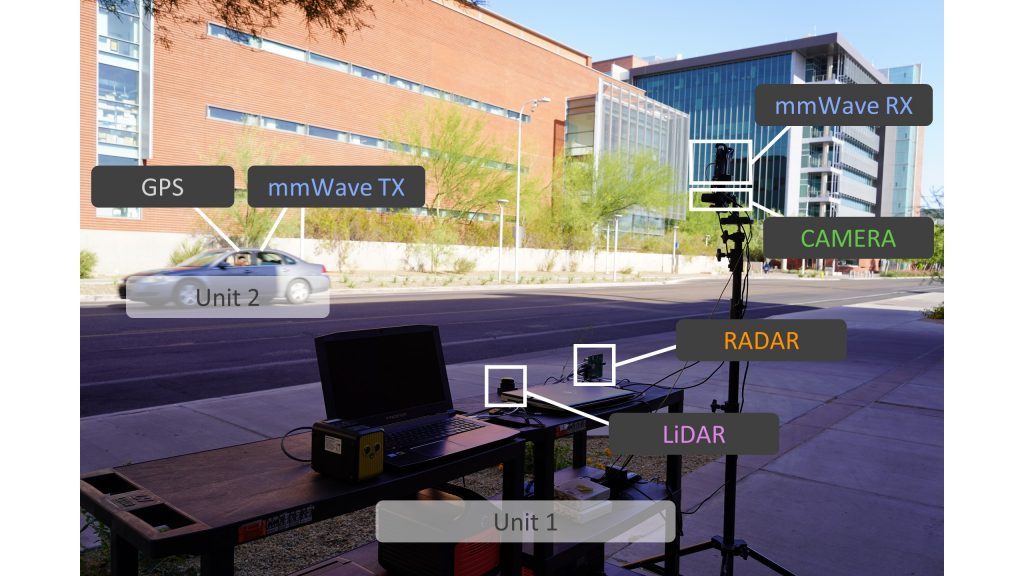
DeepSense 6G is a real-world multi-modal dataset that comprises coexisting multi-modal sensing and communication data, such as mmWave wireless communication, Camera, GPS data, LiDAR, and Radar, collected in realistic wireless environments. Link to the DeepSense 6G website is provided below.
Scenarios [24 - 29]
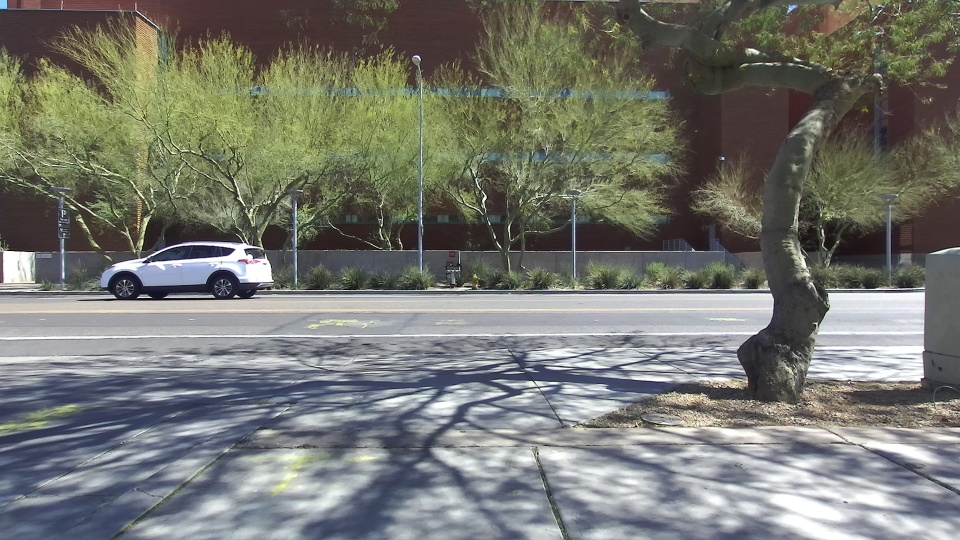
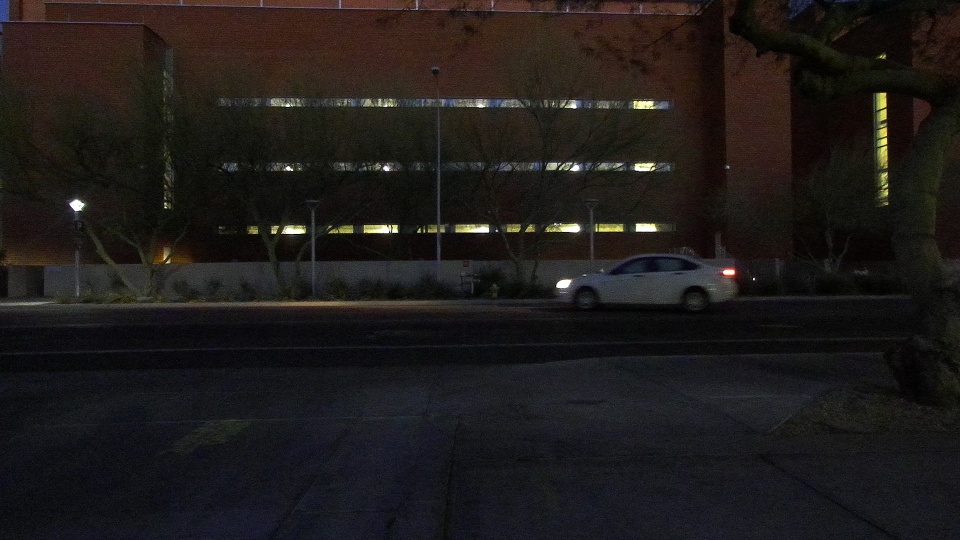
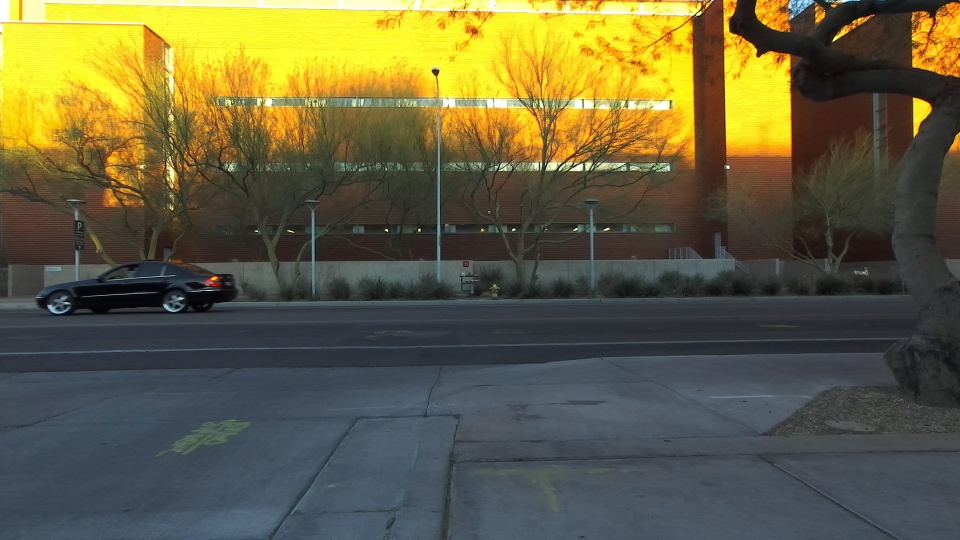
In this blockage prediction task, we build development/challenge datasets based on the DeepSense data from scenarios 24 – 29. For further details regarding the scenarios, follow the links provided below.
Citation
A. Alkhateeb, G. Charan, T. Osman, A. Hredzak, J. Morais, U. Demirhan, and N. Srinivas, “DeepSense 6G: A Large-Scale Real-World Multi-Modal Sensing and Communication Datasets,” to be available on arXiv, 2022. [Online]. Available: https://www.DeepSense6G.net
@Article{DeepSense,
author = {Alkhateeb, A. and Charan, G. and Osman, T. and Hredzak, A. and Morais, J. and Demirhan, U. and Srinivas, N.},
title = {{DeepSense 6G}: A Large-Scale Real-World Multi-Modal Sensing and Communication Dataset},
journal={to be available on arXiv},
year = {2022},
url = {https://www.DeepSense6G.net},}
S. Wu, C. Chakrabarti, and A. Alkhateeb, “LiDAR-Aided Mobile Blockage Prediction in Real-World Millimeter Wave Systems,” In Proc. of IEEE Wireless Communications and Networking Conference (WCNC), 2022.
@INPROCEEDINGS{Wu2022,
author={Wu, Shunyao and Chakrabarti, Chaitali and Alkhateeb, Ahmed},
booktitle={Proc. of IEEE Wireless Communications and Networking Conference (WCNC)},
title={LiDAR-Aided Mobile Blockage Prediction in Real-World Millimeter Wave Systems},
year={2022},
volume={},
number={},
pages={2631-2636},
doi={10.1109/WCNC51071.2022.9771651}}