Environment Semantic Communication: Enabling Distributed Sensing Aided Networks
Available on ArXiv
Shoaib Imran, Gouranga Charan, and Ahmed Alkhateeb
Wireless Intelligence Lab
Arizona State University
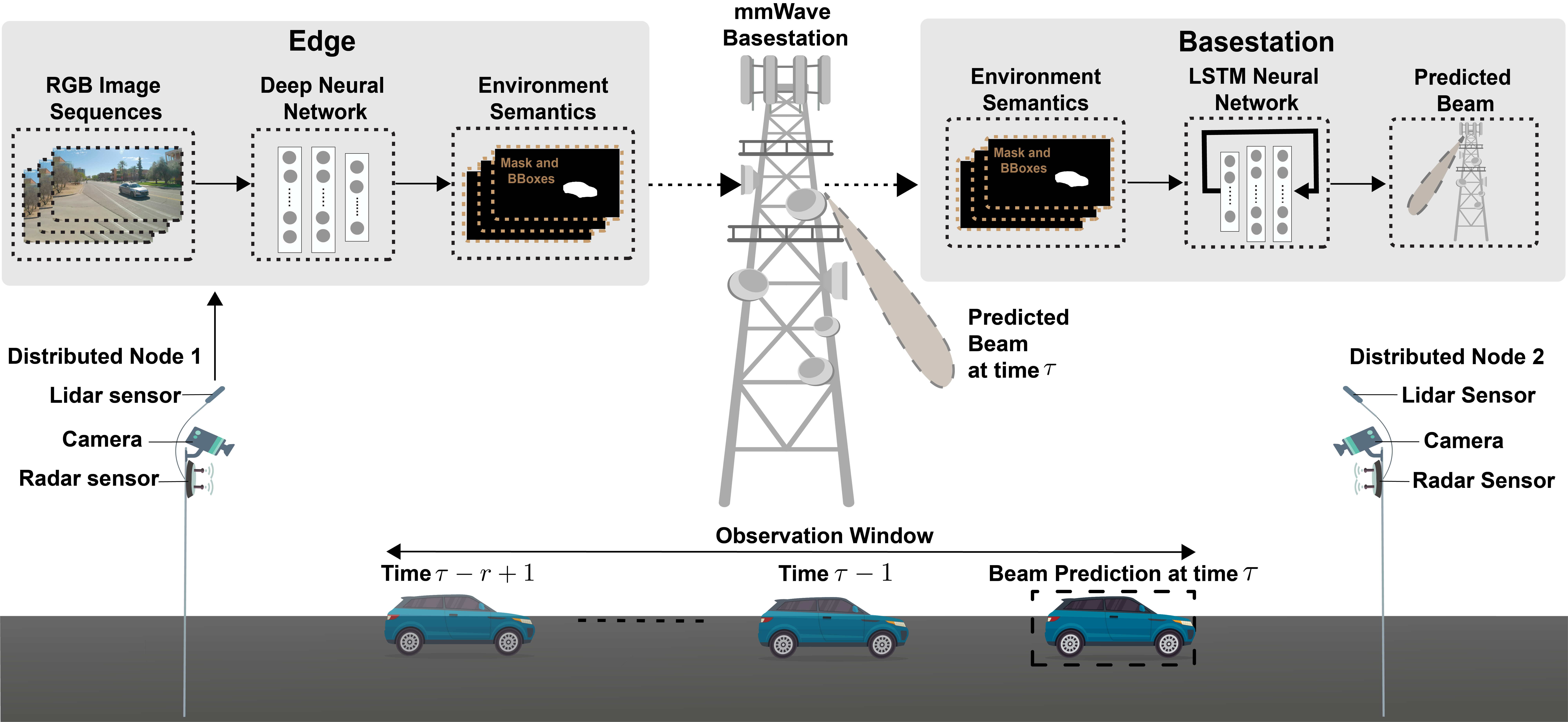
Abstract
Millimeter-wave (mmWave) and terahertz (THz) communication systems require large antenna arrays and use narrow directive beams to ensure sufficient receive signal power. However, selecting the optimal beams for these large antenna arrays incurs a significant beam training overhead, making it challenging to support applications involving high mobility. In recent years, machine learning (ML) solutions have shown promising results in reducing the beam training overhead by utilizing various sensing modalities such as GPS position and RGB images. However, the existing approaches are mainly limited to scenarios with only a single object of interest present in the wireless environment and focus only on co-located sensing, where all the sensors are installed at the communication terminal. This brings key challenges such as the limited sensing coverage compared to the coverage of the communication system and the difficulty in handling non-line-of-sight scenarios. To overcome these limitations, our paper proposes the deployment of multiple distributed sensing nodes, each equipped with an RGB camera. These nodes focus on extracting environmental semantics from the captured RGB images. The semantic data, rather than the raw images, are then transmitted to the basestation. This strategy significantly alleviates the overhead associated with the data storage and transmission of the raw images. Furthermore, semantic communication enhances the system’s adaptability and responsiveness to dynamic environments, allowing for prioritization and transmission of contextually relevant information. Experimental results on the DeepSense 6G dataset demonstrate the effectiveness of the proposed solution in reducing the sensing data transmission overhead while accurately predicting the optimal beams in realistic communication environments.
Proposed Solution
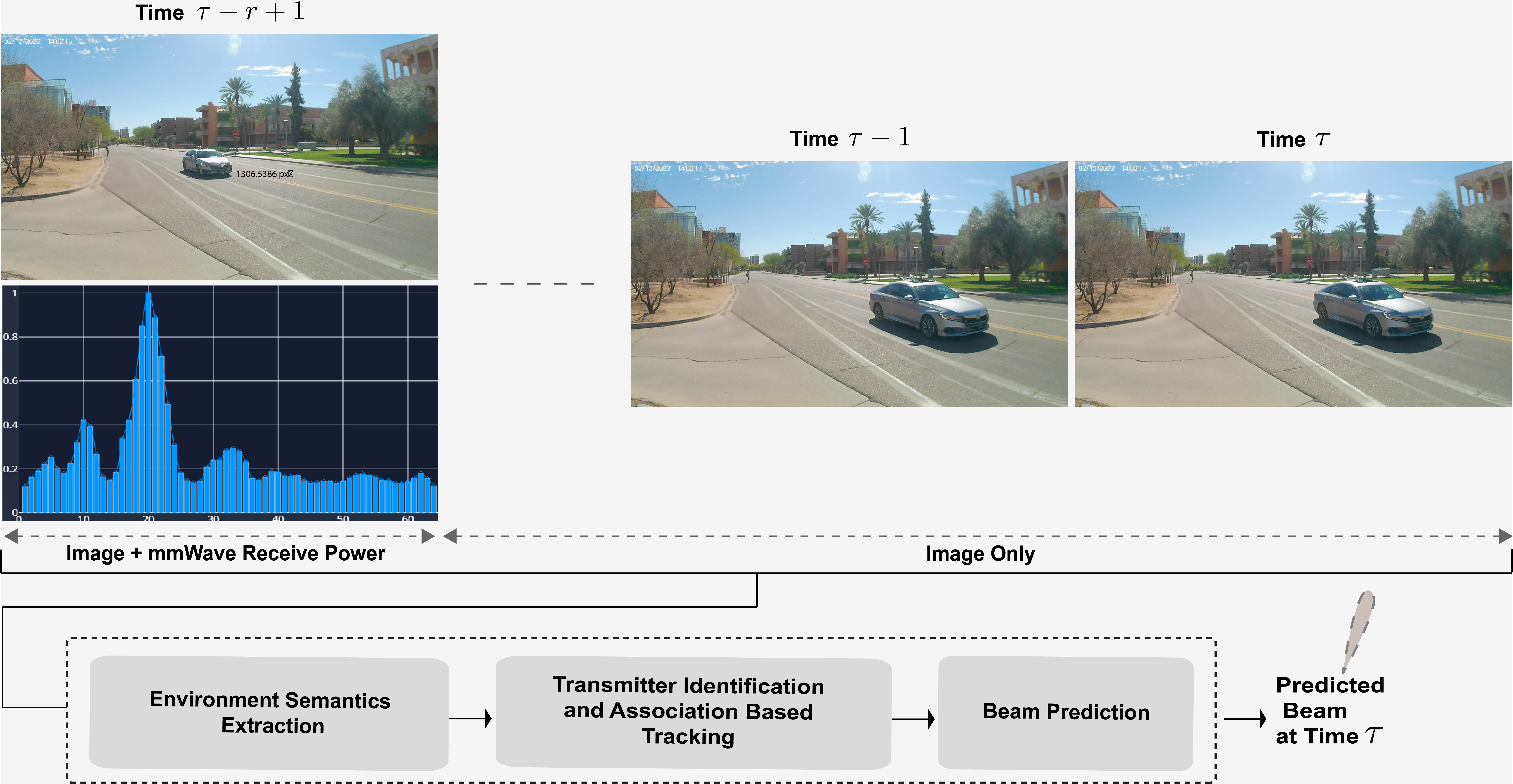
This figure outlines the different stages of the proposed solution. First, we extract environment semantics from the raw RGB images, transmitting
them to the basestation. In the second stage, we identify the transmitter in the initial frame and track it over the subsequent frames. The final step involves
using this semantic information of the transmitter, accumulated in the second stage, for beam prediction.
Video Presentation
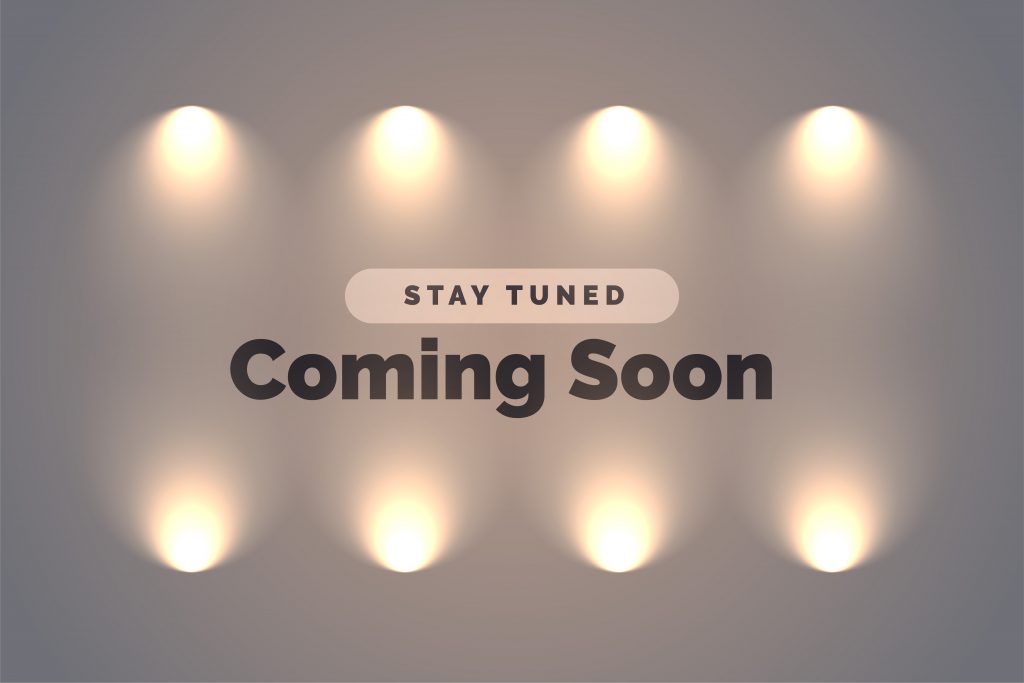
DeepSense 6G Dataset
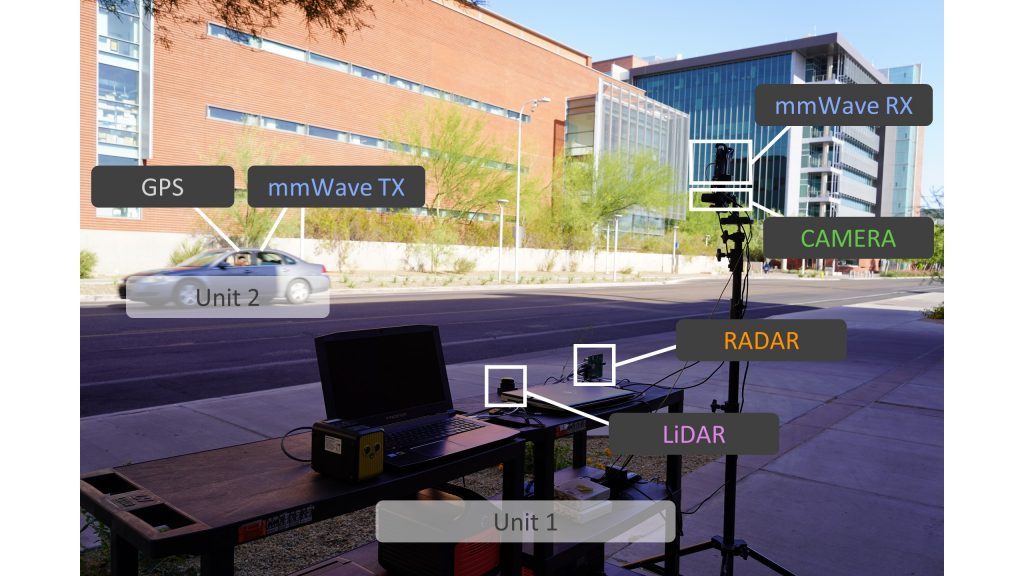
DeepSense 6G is a real-world multi-modal dataset that comprises coexisting multi-modal sensing and communication data, such as mmWave wireless communication, Camera, GPS data, LiDAR, and Radar, collected in realistic wireless environments. Link to the DeepSense 6G website is provided below.
Scenario 41
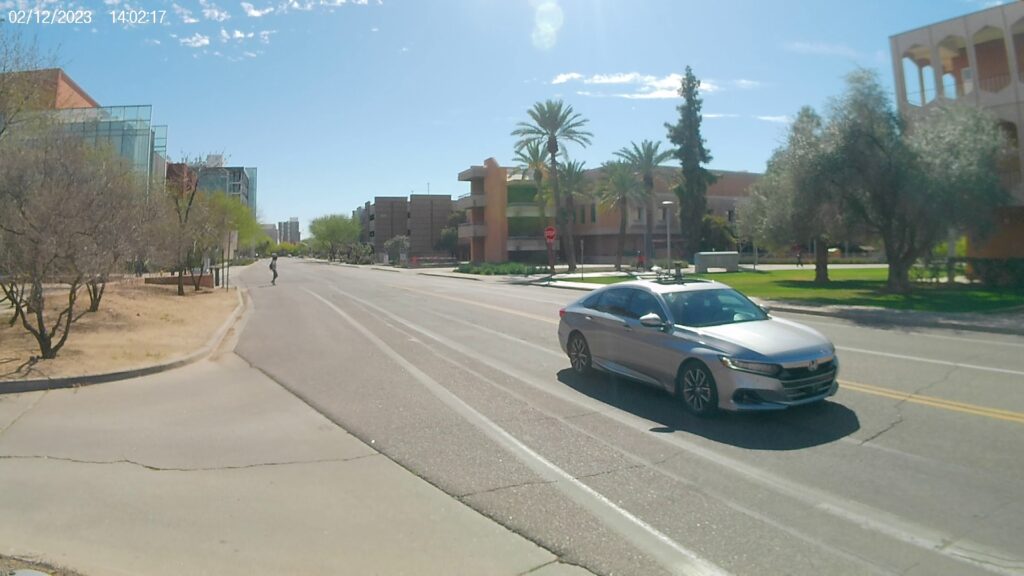
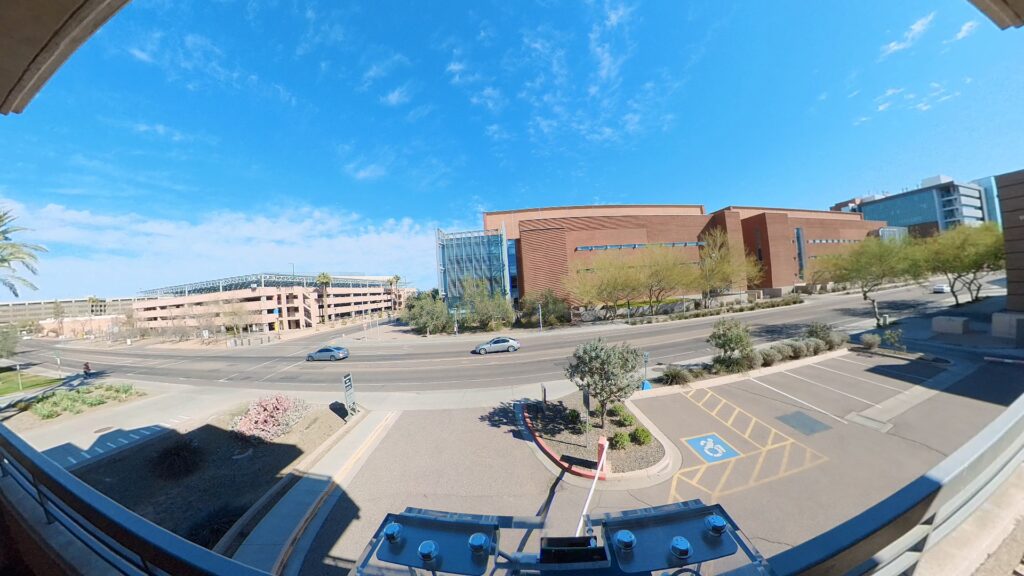
In this figure, we present the different image samples of scenarios 41
Citation
A. Alkhateeb, G. Charan, T. Osman, A. Hredzak, J. Morais, U. Demirhan, and N. Srinivas, “DeepSense 6G: A Large-Scale Real-World Multi-Modal Sensing and Communication Datasets,” IEEE Communications Magazine, 2023.
@Article{DeepSense,
author={Alkhateeb, Ahmed and Charan, Gouranga and Osman, Tawfik and Hredzak, Andrew and Morais, Joao and Demirhan, Umut and Srinivas, Nikhil},
title={DeepSense 6G: A Large-Scale Real-World Multi-Modal Sensing and Communication Dataset},
journal={IEEE Communications Magazine},
year={2023},
publisher={IEEE}}
Imran, Shoaib, Gouranga Charan, and Ahmed Alkhateeb. “Environment Semantic Communication: Enabling Distributed Sensing Aided Networks.” arXiv preprint arXiv:2402.14766 (2024).
@article{imran2024environment,
title={Environment Semantic Communication: Enabling Distributed Sensing Aided Networks},
author={Imran, Shoaib and Charan, Gouranga and Alkhateeb, Ahmed},
journal={arXiv preprint arXiv:2402.14766},
year={2024}
}