Environment Semantic Aided Communication: A Real World Demonstration for Beam Prediction
Submitted to IEEE International Conference on Communications (ICC) Workshop 2023
Shoaib Imran, Gouranga Charan, Ahmed Alkhateeb
Wireless Intelligence Lab
Arizona State University
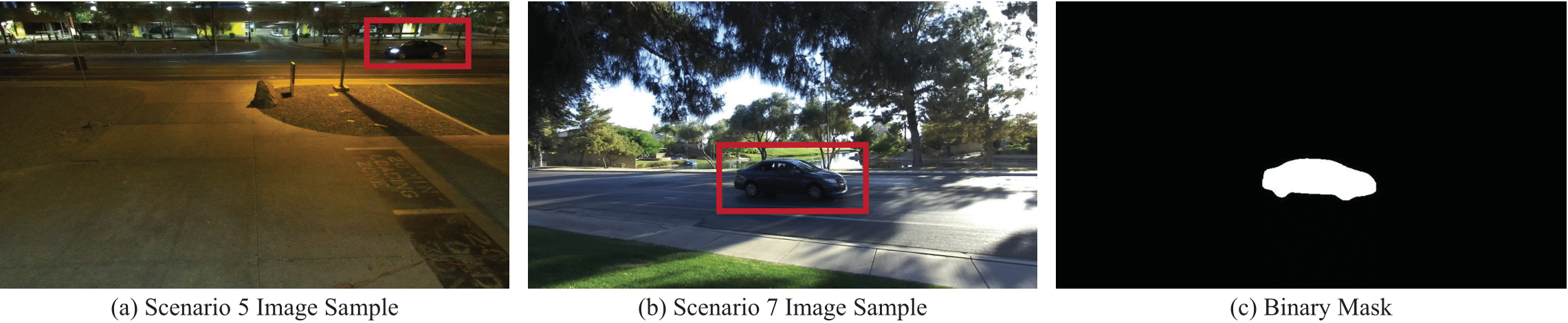
We showcase the effectiveness of the proposed solution using both masks and bounding boxes of the mobile units. Fig. (a) and (b) show the bounding box of the mobile unit on top of the RGB image for scenario 5 and 7 respectively. Fig. (c) on the other hand shows the corresponding mask of the mobile unit in the image.
Abstract
Millimeter-wave (mmWave) and terahertz (THz) communication systems adopt large antenna arrays to ensure
adequate receive signal power. However, adjusting the narrow beams of these antenna arrays typically incurs high beam training overhead that scales with the number of antennas. Recently proposed vision-aided beam prediction solutions, which utilize raw RGB images captured at the basestation to predict the optimal beams, have shown initial promising results. However, they still have a considerable computational complexity, limiting their adoption in the real world. To address these challenges, this paper focuses on developing and comparing various approaches
that extract lightweight semantic information from the visual data. The results show that the proposed solutions can significantly decrease the computational requirements while achieving similar beam prediction accuracy compared to the previously proposed vision-aided solutions.
Proposed Solution
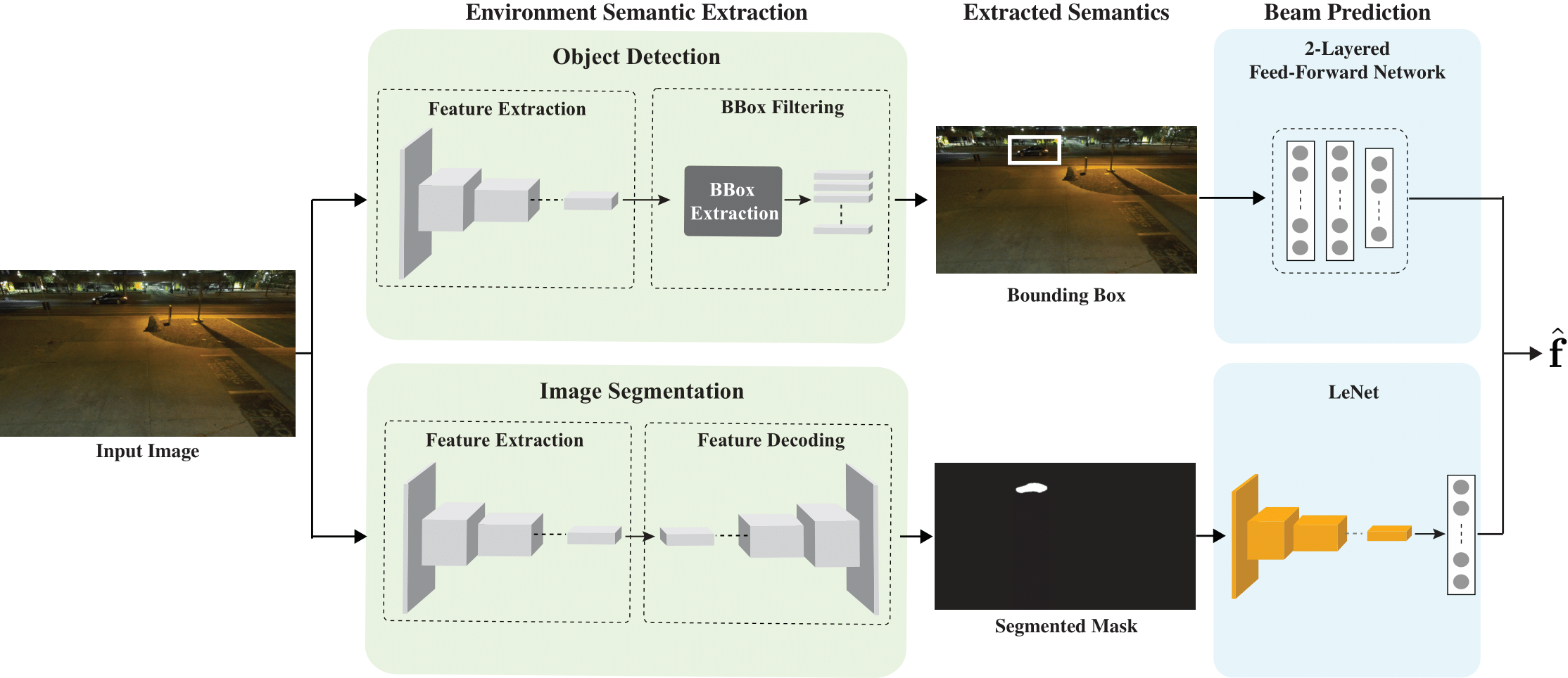
A block diagram showing the proposed solution for environment semantics-aided beam prediction task. The camera installed at the basestation captures real-time images of the wireless environment. We propose to first extract environment semantics (object masks, bounding boxes, etc.) from the RGB images. These extracted semantics can then be used to predict the optimal beam indices.
Video Demo
Reproducing the Results
DeepSense 6G Dataset
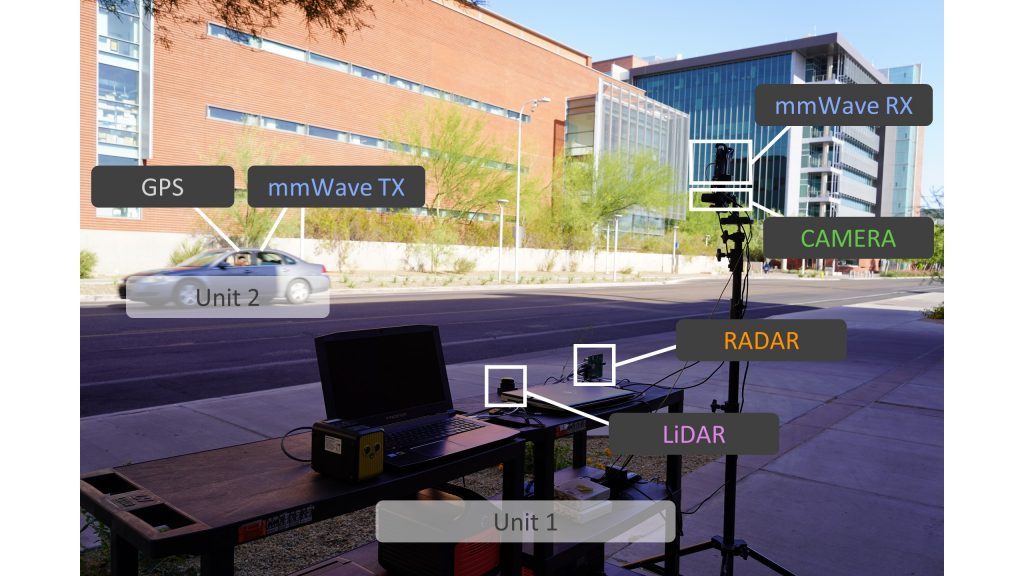
DeepSense 6G is a real-world multi-modal dataset that comprises coexisting multi-modal sensing and communication data, such as mmWave wireless communication, Camera, GPS data, LiDAR, and Radar, collected in realistic wireless environments. Link to the DeepSense 6G website is provided below.
Scenarios
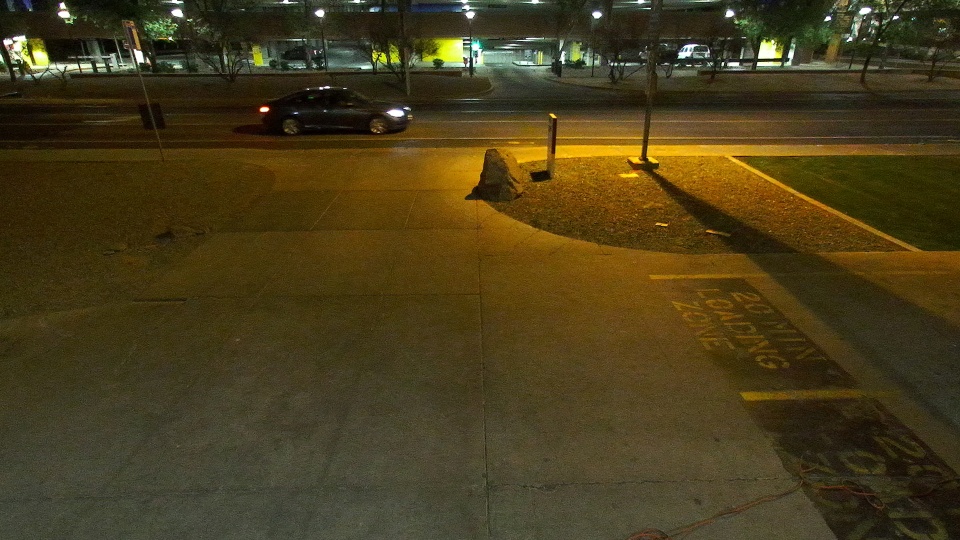
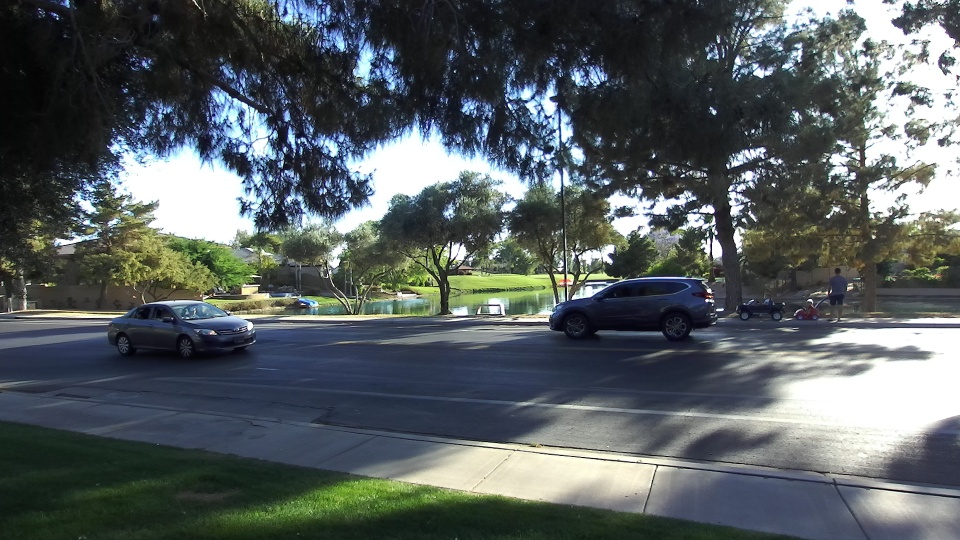
Citation
A. Alkhateeb, G. Charan, T. Osman, A. Hredzak, J. Morais, U. Demirhan, and N. Srinivas, “DeepSense 6G: A Large-Scale Real-World Multi-Modal Sensing and Communication Dataset,” arXiv preprint arXiv:2211.09769 (2022) [Online]. Available: https://www.DeepSense6G.net
@article{alkhateeb2022deepsense,
title={DeepSense 6G: A large-scale real-world multi-modal sensing and communication dataset},
author={Alkhateeb, Ahmed and Charan, Gouranga and Osman, Tawfik and Hredzak, Andrew and Morais, Jo{\~a}o and Demirhan, Umut and Srinivas, Nikhil},
journal={arXiv preprint arXiv:2211.09769},
year={2022}}
S. Imran, G. Charan and A. Alkhateeb. “Environment Semantic Aided Communication: A Real World Demonstration for Beam Prediction.” arXiv preprint arXiv:2302.06736 (2023).
@inproceedings{Imran2023EnvironmentSA,
title={Environment Semantic Aided Communication: A Real World Demonstration for Beam Prediction},
author={Imran, Shoaib and Charan, Gouranga and Alkhateeb, Ahmed,
journal={arXiv preprint arXiv:2302.06736}
year={2023}}