Digital Twin Aided Compressive Sensing:
Enabling Site-Specific MIMO Hybrid Precoding
Submitted to the Asilomar Conference on Signals, Systems, and Computers, 2024
Hao Luo and Ahmed Alkhateeb
Wireless Intelligence Lab, ASU
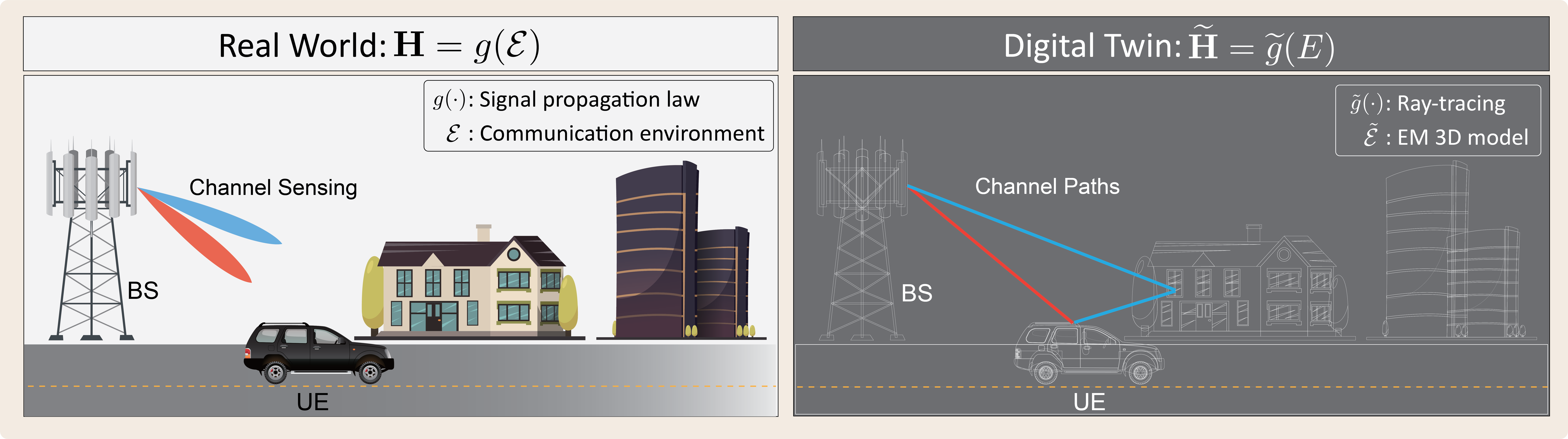
This figure shows the communication system and its digital twin. The base station is equipped with a hybrid architecture and applies compressive sensing measurement vectors for channel sensing. The measurements are then used to design hybrid precoding vectors. In this work, we propose leveraging site-specific digital twin synthetic data to train deep learning models for learning measurement vectors and hybrid precoder/combiner design in an end-to-end manner.
Abstract
Compressive sensing is a promising solution for the channel estimation in multiple-input multiple-output (MIMO) systems with large antenna arrays and constrained hardware. Utilizing site-specific channel data from real-world systems, deep learning can be employed to learn the compressive sensing measurement vectors with minimum redundancy, thereby focusing sensing power on promising spatial directions of the channel. Collecting real-world channel data, however, is challenging due to the high overhead resulting from the large number of antennas and hardware constraints. In this paper, we propose leveraging a site-specific digital twin to generate synthetic channel data, which shares a similar distribution with real-world data. The synthetic data is then used to train the deep learning models for learning measurement vectors and hybrid precoder/combiner design in an end-to-end manner. We further propose a model refinement approach to fine-tune the model pre-trained on the digital twin data with a small amount of real-world data. The evaluation results show that, by training the model on the digital twin data, the learned measurement vectors can be efficiently adapted to the environment geometry, leading to high performance of hybrid precoding for real-world deployments. Moreover, the model refinement approach can enable the digital twin aided model to achieve comparable performance to the model trained on the real-world dataset with a significantly reduced amount of real-world data.
Proposed Solution
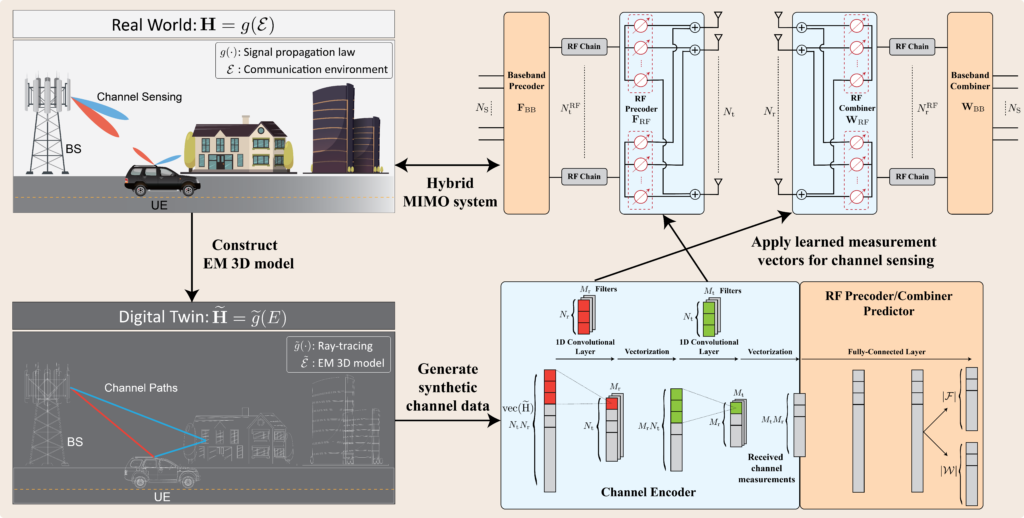
This diagram illustrates the key idea of the proposed digital twin aided compressive sensing for hybrid precoding. The digital twin generates synthetic channel data, which shares a similar distribution as the real-world data. The channel encoder mimics the channel sensing process and learns the transmit and receive measurement vectors that capture the promising spatial directions of the channel. Based on the received channnel measurements, the RF precoder/combiner predictor predicts the codebook indices for the RF precoder/combiner. The RF precoder/combiner predictor, for instance, can be implemented in the baseband processor at the receiver.
Video Presentation
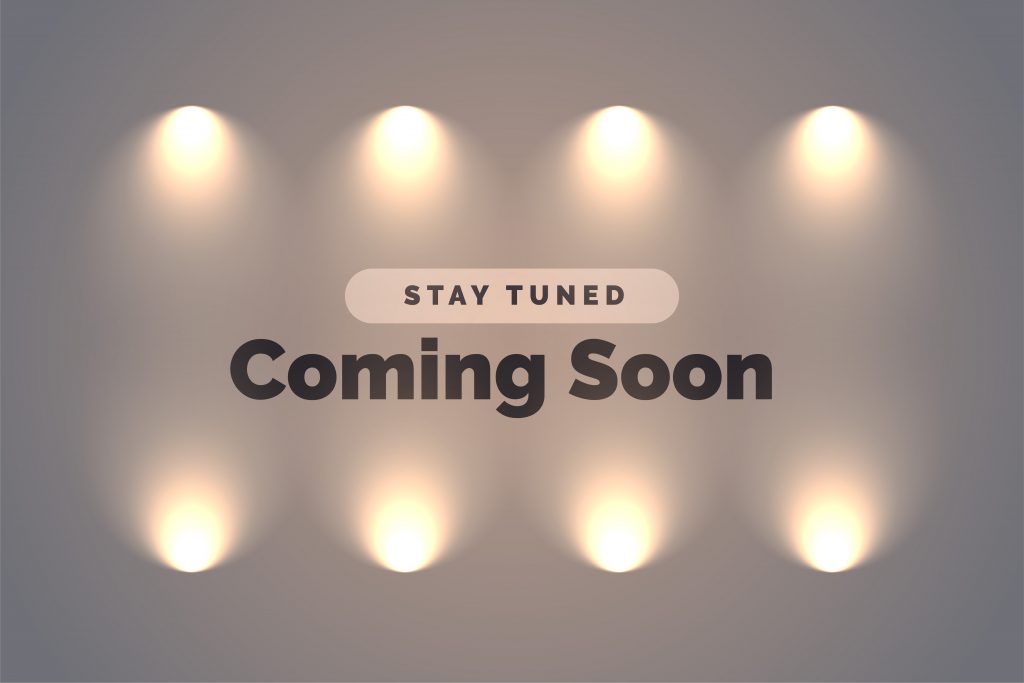
Reproducing the Results
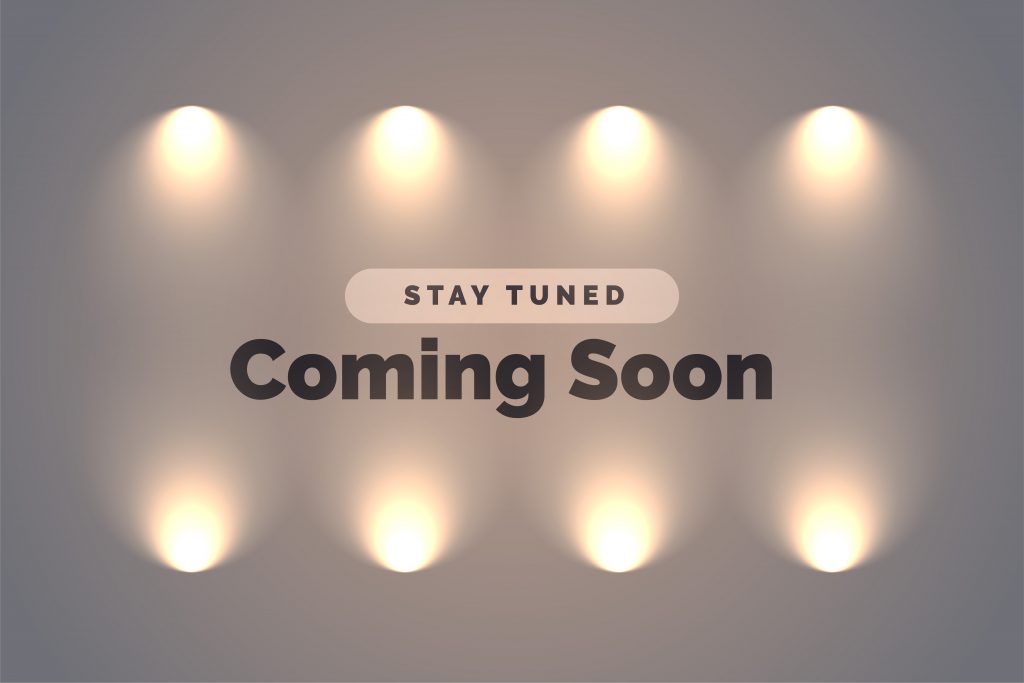
Citation
H. Luo and A. Alkhateeb. “Digital Twin Aided Compressive Sensing: Enabling Site-Specific MIMO Hybrid Precoding.” arXiv preprint arXiv:2405.07115.
@Article{Luo2024, title={Digital Twin Aided Compressive Sensing: Enabling Site-Specific MIMO Hybrid Precoding}, author={Luo, Hao and Alkhateeb, Ahmed}, journal={arXiv preprint arXiv:2405.07115}, year={2024} }