Blockage Prediction Using Wireless Signatures
Fig 1. a mmWave/sub-THz BS utilizes the received mmWave/sub-THz signal power to enable the proposed proactive dynamic link blockage prediction
Key ideas
- Any wireless environment can be broadly broken down into two categories of objects: stationary and dynamic.
- The behavior of these objects affect the behavior of the wireless channel
- The object acts like a scatterer for the signal propagating from the TX to the RX when moving in this environment till it blocks this LOS path.
- The received signal during this interval will experience a constructive and destructive interference from the LOS ray and the ray scattered on the moving object
- The contribution of the moving blockage/scatterer will change as the scatterer approaches the LOS link and before it blocks the link.
Applications
- Proactively manage hand-off/beam-switching decisions
- Reduce initial access latency, increase reliability and resource utilization efficiency of future mmWave/THz networks
More information about this research direction
Paper: Shunyao Wu, Muhammad Alrabeiah, Chaitali Chakrabarti, and Ahmed Alkhateeb, “Blockage Prediction Using Wireless Signatures: Deep Learning Enables Real-World Demonstration,” arXiv preprint arXiv:2111.08242 (2021).
Abstract: Overcoming the link blockage challenges is essential for enhancing the reliability and latency of millimeter wave (mmWave) and sub-terahertz (sub-THz) communication networks. Previous approaches relied mainly on either (i) multiple-connectivity, which under-utilizes the network resources, or on (ii) the use of out-of-band and non-RF sensors to predict link blockages, which is associated with increased cost and system complexity. In this paper, we propose a novel solution that relies only on in-band mmWave wireless measurements to proactively predict future dynamic line-of-sight (LOS) link blockages. The proposed solution utilizes deep neural networks and special patterns of received signal power, that we call pre-blockage wireless signatures to infer future blockages. Specifically, the developed machine learning models attempt to predict: (i) If a future blockage will occur? (ii) When will this blockage happen? (iii) What is the type of the blockage? And (iv) what is the direction of the moving blockage? To evaluate our proposed approach, we build a large-scale real-world dataset comprising nearly 0.5 million data points (mmWave measurements) for both indoor and outdoor blockage scenarios. The results, using this dataset, show that the proposed approach can successfully predict the occurrence of future dynamic blockages with more than 85\% accuracy. Further, for the outdoor scenario with highly-mobile vehicular blockages, the proposed model can predict the exact time of the future blockage with less than 80ms error for blockages happening within the future 500ms. These results, among others, highlight the promising gains of the proposed proactive blockage prediction solution which could potentially enhance the reliability and latency of future wireless networks.
@misc{Wu2021,
title={Blockage Prediction Using Wireless Signatures: Deep Learning Enables Real-World Demonstration},
author={Wu, Shunyao and Alrabeiah, Muhammad and Chakrabarti, Chaitali and Alkhateeb, Ahmed},
year={2021},
eprint={2111.08242},
archivePrefix={arXiv},
primaryClass={eess.SP}}
To reproduce the results in this paper:
Simulation codes:
Coming soon
These simulations use the DeepSense scenarios:
Scenario "x"
Scenario "y"
Example: Steps to generate the results in this figure
- coming soon
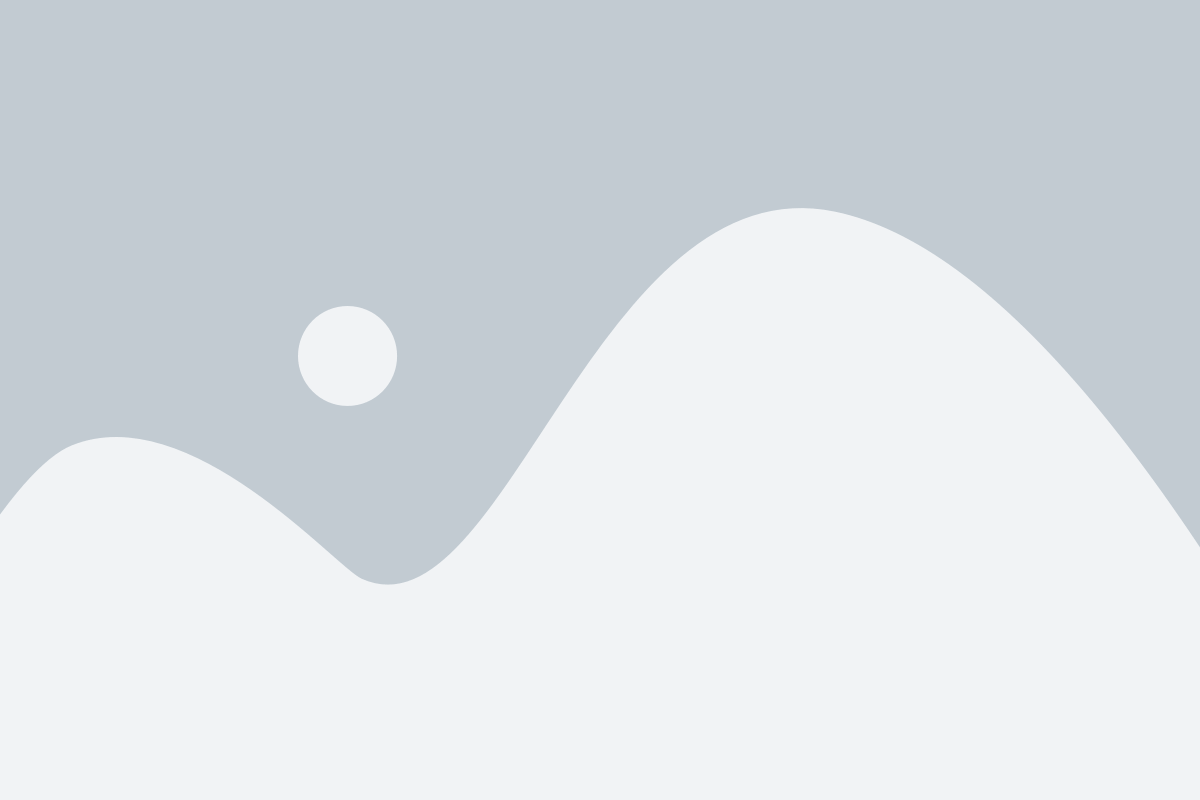